作者:A Karnewar, A Vedaldi, D Novotny, N Mitra
[Meta AI & UCL]
总结:
提出一种新的扩展扩散模型到3D图像生成的方法,只需使用2D图像作为监督来训练,使用一个可学习的渲染模块和预训练的特征编码器来解决记忆和计算复杂性的问题。
推荐说明:
- 背景:将扩散模型扩展到3D图像生成很难,主要因为缺乏大量的3D训练数据和模型的记忆和计算复杂性问题。
- 思路:提出一种新的扩散模型和图像形成模型,使用2D图像作为监督来训练,使用一个可学习的渲染模块和预训练的特征编码器来解决记忆和计算复杂性的问题。
- 价值:可扩展性好、鲁棒性强,与现有的3D生成建模方法相比,样本质量和保真度竞争力强。
https://arxiv.org/abs/2303.16509
Diffusion models have emerged as the best approach for generative modeling of 2D images. Part of their success is due to the possibility of training them on millions if not billions of images with a stable learning objective. However, extending these models to 3D remains difficult for two reasons. First, finding a large quantity of 3D training data is much more complex than for 2D images. Second, while it is conceptually trivial to extend the models to operate on 3D rather than 2D grids, the associated cubic growth in memory and compute complexity makes this infeasible. We address the first challenge by introducing a new diffusion setup that can be trained, end-to-end, with only posed 2D images for supervision; and the second challenge by proposing an image formation model that decouples model memory from spatial memory. We evaluate our method on real-world data, using the CO3D dataset which has not been used to train 3D generative models before. We show that our diffusion models are scalable, train robustly, and are competitive in terms of sample quality and fidelity to existing approaches for 3D generative modeling.
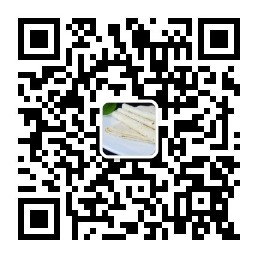