作者吴恩达,全球人工智能教育及研究领导者、DeepLearning.AI创始人
本文转自吴恩达知乎专栏
亲爱的朋友们:
上周,科技新闻网站The Information报道了谷歌的内部争议。工程师们担心谷歌的Bard大型语言模型部分是根据OpenAI的ChatGPT的输出进行训练的,这违反了OpenAI的使用条款。据称,这些输出数据是在ShareGPT网站托管的,用户可以在这个网站上与ChatGPT分享对话。(谷歌对此做出否认。)十年前,谷歌曾指责微软抄袭其搜索结果来增强必应(Bing)的性能。
在不同模型的输出上训练机器学习模型可能是一种有用的技术,但它也会引发工程、业务和法律问题。这些问题什么时候能得到解决呢?
在生成数据上训练学习算法的工程方式仍在开发中。当我领导一个大型的自动语音识别 (automatic speech recognition, ASR) 团队时,有传言说一个竞争对手正在使用我们的系统生成文本来训练竞品系统,对此我们从未证实或反驳过。据说,该竞争对手并没有直接使用我们ASR系统的输出作为标记过的训练数据,而是使用了一个轻量进程来手动清除错误,并确保数据是高质量的。
最近,我看到许多开发人员在对用例进行试验,例如prompting一个大型模型(例如175B个参数)生成专门用于应用程序(例如客户服务系统)的高质量输出,并使用此数据微调一个较小的模型(低于10B个参数),这样每次推理的成本更低。加州大学伯克利分校使用ShareGPT的数据训练了Koala ,斯坦福大学通过微调Meta的LLaMA来训练 Alpaca(这些数据是在OpenAI的text-davinci-003的帮助下生成的)。
这种做法引发了重要的商业问题。你可能花了很多精力来收集一个大的标记训练集,但竞争对手可以使用你的模型输出来获得优势。这种可能性表明,与传统的技术—商业智慧相反,数据并不总能让你的企业更有说服力。具体来说,如果一家市场领导企业花费大量资源使其性能达到一定水平,并且如果其产品生成的数据使竞争对手追赶起来的成本更低,那么其最初花费在收集数据上的努力就无法对竞争对手产生强有力防御。
此外,围绕这种做法的法律和伦理问题需要更明确的答案。OpenAI的使用条款禁止任何人“使用服务的输出来开发与OpenAI竞争的模型”。在我看来,这引发了如下法律问题:
● 如果谷歌或其他公司没有同意OpenAI的使用条款,并且从其他人共享的ShareGPT中抓取文本,它是否会受OpenAI的条款约束?
● 根据反垄断法和公平使用法,“限制竞争对手使用你的服务”的条款是否真的具有可执行性?
(当然我不并是律师,请不要把我说的任何话理解为法律建议。)
在生成式人工智能时代,我们将看到许多针对使用一个模型生成数据来训练另一个模型的创造性用例。这是一个令人兴奋的技术趋势,但我们需要牢记以合法和公平的方式向前发展。
请继续进行微调!
吴恩达
Dear friends,
Last week, the tech news site The Information reported an internal controversy at Google. Engineers were concerned that Google’s Bard large language model was trained in part on output from OpenAI’s ChatGPT, which would have violated OpenAI’s terms of use. The output purportedly was hosted on ShareGPT, a website where users share conversations with ChatGPT. (Google denies the report.) A decade ago, Google accused Microsoft of copying its search results to enhance Bing.
Training a machine learning model on a different model’s output can be a useful technique, but it also raises engineering, business, and legal questions. When is it okay?
Engineering recipes for training learning algorithms on generated data are still being developed. When I led a large automatic speech recognition (ASR) team, there were rumors — that we never proved or disproved — that a competitor was using our system to generate transcripts to train a competing system. It was said that, rather than using our ASR system’s output directly as labeled training data, our competitor used a lightweight process to manually clean up errors and make sure the data was high-quality.
Lately, I’ve seen many developers experiment with use cases such as prompting a large model (say, 175B parameters) to generate high-quality outputs specialized to an application such as customer support, and using this data to fine-tune a smaller model (say, ~10B parameters) that costs less per inference. UC Berkeley trained Koala using data from ShareGPT, and Stanford trained Alpaca by fine-tuning Meta’s LLaMA on data generated with assistance from OpenAI’s text-davinci-003.
Such recipes raise important business questions. You may have spent a lot of effort to collect a large labeled training set, yet a competitor can use your model’s output to gain a leg up. This possibility argues that, contrary to conventional tech-business wisdom, data doesn’t always make your business more defensible. Specifically, if a market leader spent significant resources to get its performance up to a certain level, and if the market leader’s product generates data that makes it cheaper for competitors to catch up, then the market leader’s initial effort spent gathering data is a weak defense against competitors.
In addition, the legal and ethical questions around this practice need clearer answers. OpenAI’s terms of use forbid anyone to “use output from the Services to develop models that compete with OpenAI.” To my mind, this raises legal questions such as:
- If Google or another company has not agreed to OpenAI’s terms of use, and it scrapes text from ShareGPT that someone else shared, is it bound by OpenAI’s terms?
- Are terms that restrict competitor’s access to your services enforceable in light of antitrust and fair-use laws?
(To state the obvious, I am not a lawyer. Don’t construe anything I say as legal advice!)
In the era of generative AI, we’ll see many creative use cases for intentionally using one model to generate data to train another. This is an exciting technical trend, even as we keep in mind the need to move forward in ways that are legal and fair.
Keep fine-tuning!
Andrew
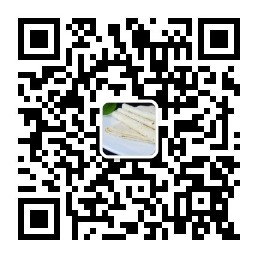