

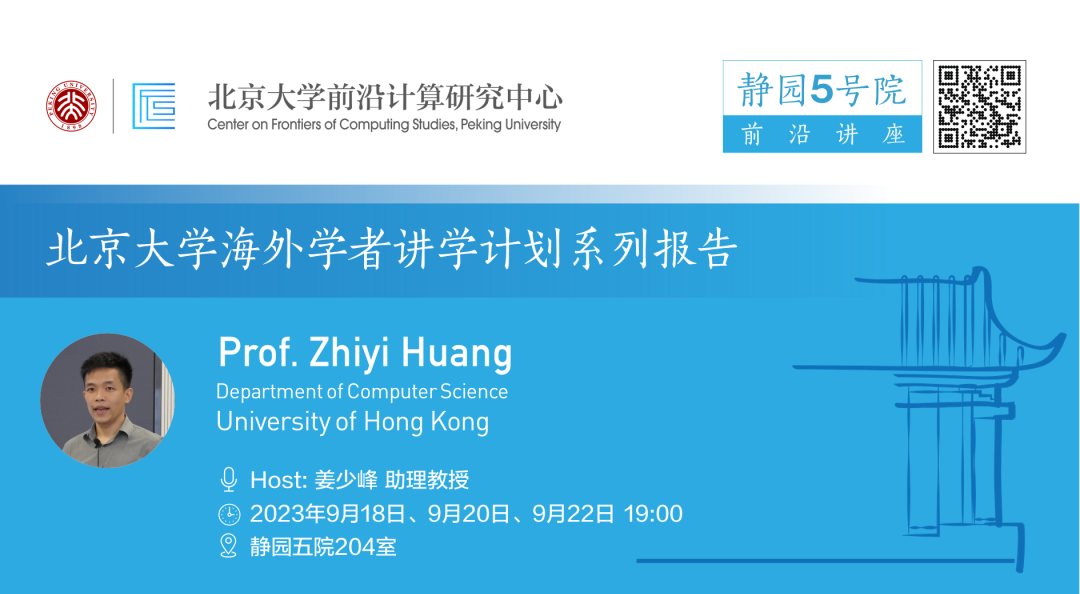
北京大学海外名家讲学计划
系列报告
报告人
Prof. Zhiyi Huang
Department of Computer Science
University of Hong Kong
报告地点
静园五院204
Host
姜少峰 助理教授
报告一
Data-driven Auction Design I: Model and Basic Techniques
时间
2023年9月18日 19:00
Abstract
The celebrated optimal auction by Myerson (1981) relies on fine-grained knowledge of the bidders’ value distributions. In reality, we can at best estimate such distributions from the available data. Is it possible to find a near-optimal solution despite the uncertainty of the distribution? If so, how much data is needed?
This is the first part of a three-lecture series on data-driven auction design. We will first introduce the sample complexity model for auction design proposed by Cole and Roughgarden (2014). Then, we will cover some basic tools, including several standard inequalities from statistics and information theory. Finally, we will explain how to use these basic tools to determine the optimal sample complexity (up to logarithmic factors) for optimal auction/pricing in the simplest single-item single-bidder case.
报告二
Data-driven Auction Design II: Progress via Statistical Learning Theory
时间
2023年9月20日 19:00
Abstract
The sample complexity of optimal auctions is a special case of the generalization problem in general learning (see, for example, the textbook formulation by Vapnik, 1998). Therefore, researchers have turned to statistical learning theory to understand the sample complexity of auctions. Conventional wisdom from learning theory asserts that the sample complexity is determined by the “degree of freedom” of the solution space, which in this case is the set of all possible auctions.
The second part of the lecture series will present a direct proof of an upper bound for the “degree of freedom” of single-item, multi-bidder auctions. As applications, we will derive sample complexity upper bounds for the single-item multi-bidder case. We will also mention several metrics for “degree of freedom” from learning theory that yield essentially the same bounds. Finally, we will explain how to extend the basic techniques from part one to establish lower bounds for the single-item, multi-bidder case. However, the upper and lower bounds will differ by a polynomial factor. We will have to wait for part three to resolve this gap and to further generalize the results to all single-parameter auctions and beyond.
报告三
Data-driven Auction Design III: Learnability of Product Distributions and Strong Revenue Monotonicity
时间
2023年9月22日 19:00
Abstract
The space of distributions and the space of solutions are equally important in the original formulation of the general learning problem. However, most subsequent research on the generalization of learning problems has focused on the complexity of the solution space, while allowing the distribution space to be arbitrarily complicated. In the auction problem, the bidders’ value distributions are independent (otherwise Myerson’s auction is no longer optimal in the first place). Is the optimal sample complexity of auctions determined by the complexity/simplicity of the distribution space rather than the solution space?
The last part of this lecture series will introduce two new techniques to answer the above question affirmatively. We will show that product distributions over finite supports are learnable using polynomially many samples, and we will characterize the tight sample complexity for learning them with respect to standard metrics for distributions. Next, we will introduce the concept of strong revenue monotonicity of auctions, which naturally suggests a new notion of “distance” between value distributions tailored for the auction problem. Finally, we will explain how to use the new techniques to determine the sample complexity of all single-parameter auctions and outline how they yield tight or state-of-the-art sample complexity for multi-parameter auctions, optimal stopping problems, etc.
报告人简介
Zhiyi is an associate professor of Computer Science at the University of Hong Kong. He works broadly on Theoretical Computer Science and Algorithmic Game Theory, with a focus on optimization and decision-making under uncertainty. Before joining HKU, Zhiyi was a postdoc at Stanford University from 2013 to 2014, working with Tim Roughgarden. He obtained his Ph.D. from the University of Pennsylvania under Sampath Kannan and Aaron Roth in 2013. During grad school, Zhiyi interned at Microsoft Research Redmond under Nikhil R. Devanur in the summers of 2011 and 2012. Before that he got a bachelor degree from the first “Yao Class” under Andrew Yao at Tsinghua University in 2008. Zhiyi was the recipient of the Best Paper Awards of FOCS 2020 and SPAA 2015, an Excellent Young Scientists Fund (HK & Macau) by NSFC, an Early Career Award by RGC Hong Kong, and a Morris and Dorothy Rubinoff Dissertation Award.
往 期 讲 座
— 版权声明 —
本微信公众号所有内容,由北京大学前沿计算研究中心微信自身创作、收集的文字、图片和音视频资料,版权属北京大学前沿计算研究中心微信所有;从公开渠道收集、整理及授权转载的文字、图片和音视频资料,版权属原作者。本公众号内容原作者如不愿意在本号刊登内容,请及时通知本号,予以删除。
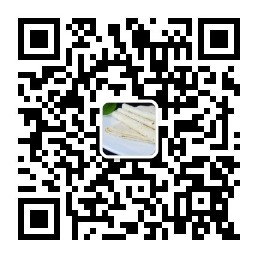