Replacing softmax with ReLU in Vision Transformers
M Wortsman, J Lee, J Gilmer, S Kornblith
[Google DeepMind]
在视觉Transformer中将Softmax替换为ReLU
- 探索用点积激活函数(如ReLU)替换transformer注意力机制中的softmax,之前的工作发现,这种改变会降低准确率。
- 关键发现是,通过序列长度除以点积激活函数(如ReLU),可以减轻与softmax注意力相比的准确率损失。
- 在ImageNet上的视觉transformer实验表明,除以序列长度的ReLU注意力可以达到softmax注意力的准确率。
- ReLU注意力允许在序列长度上并行化, Gather操作较少于softmax注意力。
- 文章假设序列长度缩放可以维持期望的注意力权重量级,在初始化时,期望的softmax权重量级为O(1/L)。
动机: 之前的研究发现,在将注意力softmax替换为ReLU等点对点激活时,准确率会降低。在视觉Transformer中,本文发现通过除以序列长度可以减轻这种降级。实验表明,在ImageNet-21k上训练小到大的视觉Transformer时,ReLU-attention可以在计算方面达到或接近softmax-attention的性能。 方法:探索了替代softmax操作的点对点方法,这些方法不一定输出概率分布。特别地,观察到通过序列长度除以ReLU的注意力可以在计算方面达到或接近传统softmax注意力的性能。这为并行化提供了新的机会,因为相比传统注意力,ReLU-attention在序列长度维度上可以进行更少的gather操作。 优势:提出了一种替代softmax的点对点注意力机制,通过除以序列长度可以减轻准确率降低的问题,并提供了更好的计算性能和并行化机会。
提出一种用ReLU替换softmax的点对点注意力机制,通过除以序列长度可以减轻准确率降低的问题,提供了更好的计算性能和并行化机会。
Previous research observed accuracy degradation when replacing the attention softmax with a pointwise activation such as ReLU. In the context of vision transformers, we find that this degradation is mitigated when dividing by sequence length. Our experiments training small to large vision transformers on ImageNet-21k indicate that ReLU-attention can approach or match the performance of softmax-attention in terms of scaling behavior as a function of compute.
https://arxiv.org/abs/2309.08586
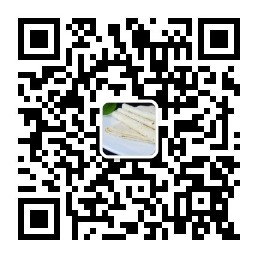