导语
大规模数据集的出现,使得追踪“科学是怎样运作”成为可能,这激励了来自不同学科背景的研究人员将科学研究的焦点转向科学本身,从而催生了迅速发展的“科学学(Science of Science)”。科学学是针对科学进行整体研究的综合性科学,具备跨学科特征,并且表现为科学观点、科学论文、科学家等尺度不同而相互关联的层次,因而其天然具备复杂系统的特征。最近发表于Nature Human Behaviour杂志的综述文章,从数据、测量和实证方法的角度,审视了“科学学”这一日益增长的跨学科领域的前沿文献。综述探讨了主要实证方法的目标、优势和局限性,以期深化对科学学领域多元化研究方法的理解,并为研究人员提供更多的研究工具。总体而言,新的实证研究方法为我们提供了强大的能力,可以检验关于科学的传统观念和理论框架,发现影响科学生产力的因素,预测科学研究的结果,并制定出有助于推动科学进步的政策。
研究领域:科学学,科研,科学方法
Lu Liu, Benjamin F. Jones, Brian Uzzi & Dashun Wang | 作者
刘培源 | 译者
论文来源:
Data, measurement and empirical methods in the science of science
论文地址:
https://www.nature.com/articles/s41562-023-01562-4
目录一、背景二、数据三、测量四、经验方法五、展望
一、背景
科学进步是提高生活水平、健康状况以及社会应对重大挑战(从气候变化到COVID-19大流行[1,2,3])的关键因素。对“科学怎样运行”和“创新在哪里发生”的更深入理解,可以帮助我们更有效地设计科学政策和科学机构,更好地为科学家自身的研究选择提供信息,并为科学和人类创造和捕获巨大价值。基于这些关键前提,近年来,“科学学”(Science of Science)领域取得了重大发展[4,5,6,7,8,9],利用大规模数据集和多样化的计算工具包揭示科学产出和科学应用背后的基本模式。
将科学方法本身作为研究对象的想法由来已久。自20世纪中叶以来,来自不同学科的研究者们一直在探讨关于科学进步的本质,以及科学研究的实践、组织和影响等核心问题。基于这些丰富的历史根源,科学学领域汲取了许多学科的知识,包括信息科学、社会科学、物理科学、生物科学、计算机科学、工程学和设计学等。科学学与元科学、科学计量学、科学经济学、研究方法学、科学技术研究、科学社会学、元知识和定量科学研究等多个研究领域和学术群体,有着紧密的联系[5]。这些群体之间存在一些明显的差异,主要体现在它们的历史起源和最初的学科组成上。例如,元科学(metascience)起源于临床科学和心理学,主要关注严谨性、透明度、可重复性以及其他与开放科学相关的实践和主题。科学计量学社区起源于图书馆和信息科学,特别强调发展稳健和负责任的科学度量和指标。科学技术研究(Science and technology studies)关注科学技术的历史、科学哲学以及科学、技术和社会之间的相互作用。科学学则起源于物理学、计算机科学和社会学,采用数据驱动的方法,强调探讨科学是如何运作的。这些学科都为理解科学做出了基础性的贡献。尽管它们在起源上存在差异,但与理解“科学实践及其对社会的影响”这一共同兴趣相比,这些差异显得微不足道。
三大领域的发展推动了科学学的快速进步。首先是“数据”的发展[9]:现代数据库汇集了数百万篇的研究论文、资助提案、专利等。这些海量的数据以前所未有的细节和规模,描绘出科学活动的全貌。其次是“测量”的发展:学者们借助这些数据,开发出许多新的科学活动度量标准,并对一些长期被视为重要但难以量化的理论进行了研究。最后是“经验方法”的发展:得益于数据科学、网络科学、人工智能和计量经济学的同步发展,研究人员现在能够以全新的方式研究关系、进行预测和评估科学政策。数据、测量和方法的革新,共同揭示了关于科学内部运作和科学进展本身的基础性新见解。
然而,多元化的方法也带来了一项主要挑战。研究者们遵循各自学科内受尊重的规范,因此其方法各异,研究成果常常发表在读者群不重叠的刊物上,导致相关研究在学科边界上呈现碎片化。这种碎片化挑战了研究者理解和欣赏自己学科以外工作价值的能力,更不用说在此基础上进行进一步探索了。
鉴于上述挑战和科学学领域的快速发展,本文回顾了该领域文献中普遍存在的实证方法。我们的目标是为读者提供关于可用数据集、测量构造和实证方法的最新理解,以及每种方法的优势和局限性。由于篇幅限制,本综述并未详尽每种方法的全部技术细节,建议读者参考相关指南以获取更多信息。相反,我们将重点解释为何研究者可能会根据研究问题,偏好选择某一种方法而非其他。
科学学的一个关键目标是,在提供对科学的超越性理解外,为科学政策提供参考。尽管本综述主要关注实证方法,其核心读者是该领域的研究者,但所梳理的研究也与关键的政策问题息息相关。例如,科学投资的适当规模是多少,应该通过哪些机构并朝哪个方向进行[10,11]?公共对科学的投资是否与公众利益相符[12]?什么条件能产生创新性或高影响力的科学[13,14,15,16,17,18,19,20]?科学的奖励体系如何影响科学进步的速度和方向[13,21,22,23,24],科学的可重复性又由什么决定[25,26,27]?科学家的贡献如何随着科学事业的发展而演变[28,29,30,31,32]?科学家的多样性如何推动科学进步[33,34,35]?以及其他与科学政策相关的问题[36,37]。
总体而言,本综述旨在促进研究人员进入科学学领域,扩展研究人员的工具包,并说明多样化的研究方法如何为我们对科学的共同理解做出贡献。第二部分回顾了数据集和数据链接。第四部分回顾了科学学中的主要测量构建。第四部分考虑了一系列经验方法,重点介绍每种方法的一个研究,并简要总结相关的例子和应用。第五部分对科学学的前景进行了总结。
二、数据
历史上,科学活动的数据收集困难,且数量有限。收集数据可能涉及从出版物中手动统计数据[38,39],采访科学家[16,40],或者整理历史轶事和传记[13,41]。分析通常局限于特定领域或科学家群体。然而,如今,大规模的科学生产和使用数据触手可得[42,43,44]。凭借大数据和先进的算法,研究人员现在可以探索以前无法量化的问题,并在范围和规模上实现了巨大的增长,如下文所述。
出版物数据集涵盖了几乎所有科学门类的论文,使得可以分析通用和特定领域的模式。常用的数据集包括Web of Science (WoS),PubMed,CrossRef,ORCID,OpenCitations,Dimensions和OpenAlex。还有一些数据集包含了论文的文本(CORE)[45,46,47],数据实体(DataCite)[48,49]和同行评审报告(Publons)[33,50,51]。这些数据集进一步支持新的测量,例如,表示论文的内容[52,53],新颖性[15,54]和跨学科性[55]。
值得注意的是,当今的数据库捕获了科学的更多元化方面,不仅仅是出版物,还提供了对研究背景和研究者自身的更丰富和更全面的视角(图1)。例如,一些数据集追踪研究资金到这些投资支持的具体出版物[56,57],这使得可以进行针对科研资金对科学生产力和公共投资回报的影响的大规模研究。包含就业情况[58,59],简历[21,59]和科学奖项[23]的数据集提供了关于科学的社会结构的丰富定量证据。将出版物概况与导师谱系[60,61],论文[34]和课程大纲[62,63]结合,可以获得关于导师指导和人才培养的见解。
图1 科学学数据及其联系。这个图展示了科学学中常用的数据类型,每种数据类型中包含的信息以及数据来源的示例。科学学中的数据集不仅规模不断增长,而且已经扩展到了出版物之外,还整合了上游的资金投资和下游的应用,超越了科学本身。
最后,当今的数据范围已经超越了科学,扩展到了社会的更广泛的方面。替代性指标(Altmetrics)[64]捕捉到新闻媒体和社交媒体上对科学文章的提及。其他数据库纳入了科学在市场上的应用,包括通过专利[10],制药临床试验和药物批准[65,66]。政策文件[67,68]帮助我们理解科学在政府各部门[69]和政策制定[12,68]中的角色。
随着当今的数据集规模越来越大,并继续整合新的维度,它们提供了以新方式揭示科学内部运作和外部影响的机会。它们可以使研究者在进行关于科学的新问题和长期问题的原创研究时,超越以往的限制。
三、测量
在这里,我们讨论科学学中突出的测量方法,包括它们的目的和局限性。
(一)引用
现代出版数据库通常包含关于哪些文章和作者引用了其他论文和科学家的数据。这些引用关系已被用于参与科学学研究的核心概念讨论。我们将考虑两种基于引用信息的常见测量方法:引用计数和知识流动。
首先,引用计数常被用作衡量影响力的指标。“指标”这个词暗示了它只是一种近似表达的概念。引用计数被定义为一个文档被后续文档引用的次数,可以作为衡量研究论文[75,76]以及专利发明重要性[77,78,79]的代替物。与平等对待每个引用不同,一些度量方法可能进一步加权考虑每个引用的重要性,例如使用引用网络结构来产生中心性[80]、PageRank[81,82]或Eigenfactor[83,84]指标。
然而,基于引用的指标也面临着批评[84,85]。引用指标必然过于简化对影响力的构造与衡量,经常忽视特定引用的含义和使用的异质性,领域和机构背景中引用实践的变化,以及科学中的声誉和权力结构对引用行为的潜在影响[86,87]。研究人员已经开始理解更为细微的引用行为,从负面引用[86]到引用上下文[47,88,89]。理解引用实际衡量了什么,在解释和应用科学学研究中的许多研究发现时非常重要。依赖于基于引用的指标而不是专家判断的评估方式,引发了指标滥用的问题[90,91,92]。鉴于开发可靠地量化和评估科学的指标的重要性,科学计量学社区一直在努力提供负责任的引用实践和评估指导[85]。
其次,科学家利用引用来追踪知识流动。论文中的每个引用都是对特定先前工作的链接,我们可以借此推测新发现是如何借鉴现有思想[76,93],以及知识如何在科学领域[94,95]、研究机构[96]、地区和国家[97,98,99],以及个体[81]之间流动。引用链接的组合也可以近似表示创新性[15]、颠覆性[17,100]和跨学科性[55,95,101,102]。一个迅速崛起的研究领域,会进一步研究来自其他领域(例如专利、临床药物试验和政策文件)对科学文章的引用,以理解科学的应用价值[10,12,65,66,103,104,105]。
(二)个体
通过对个体职业的分析,研究人员能够解答如下问题:我们应如何衡量个体的科学生产力?典型的职业生命周期是怎样的?资源和荣誉是如何在个体和职业间分配的?学者的职业生涯可以通过其发表论文 [30,31,106,107,108]来探究,其中重点关注的是职业发展与流动性、发表论文的数量及其引用影响,以及他们所获得的资助[24,109,110]和奖项[111,112,113]。
个体影响力的研究主要关注产出,通常以研究者发表的论文数量和引用指标作为近似度量。h指数[114]是衡量个体影响力的一种常用方法,它综合考虑了论文数量和每篇论文的影响力。具体来说,一个科学家的h指数是他们至少有h篇论文被引用了h次以上,这一最大数值h即为h指数。后续研究在h指数的基础上,提出了各种变体以解决其局限性[115],这些变体包括强调职业生涯中高引用论文的重要性[116],考虑领域差异[117]和标准化处理[118],以及评估个体在合作作品中的相对贡献比例[119]。
为了探究职业生涯周期中产出的动力学变化,我们可以根据个体的年龄、职业年龄或发表论文的顺序进行研究。有大量的文献研究探讨了年龄与杰出成就之间的关系[28,106,111,120,121]。近期的研究进一步解开了年龄、发表论文数量和每篇论文引用次数之间的关系,并衡量了在个人作品序列中产出高引用论文的可能性[30,31]。
尽管听起来简单,但使用出版记录来描述职业生涯却是一项挑战。收集研究者的全部出版物列表,是研究个体的基础,但这仍是一个主要挑战,需要使用姓名消歧技术将特定作品与特定研究者匹配。尽管算法在识别数百万个职业档案方面越来越有能力[122],但它们在准确性和稳健性上各不相同。ORCID数据集可以通过为研究者提供创建、维护和更新个人档案的机会来缓解这个问题,并且它不仅收集出版物,还收集更广泛的产出和活动[123]。第二个挑战是幸存偏差。实证研究往往关注那些足够长,可以进行统计分析的职业生涯,这限制了研究结果对各类科学职业的适用性。第三个挑战是科学家活动的广度,专注于出版物会忽视其他重要贡献,如指导和教学、服务(例如审稿、审查拨款提案和编辑期刊文章)或在组织内的领导地位。尽管研究者已经开始通过将个人出版物档案与学术谱谱系数据库[61,124]、论文[34]、资助[109]、个人简历[21]和致谢[125]进行关联来探索这些维度,但出版记录之外的科学职业仍然缺乏研究[126,127]。最后,基于引用的指标只能作为个人表现的近似,具有与上述讨论相似的局限性。科学界已经呼吁采取更合适的做法[85,128],从包括专家评估研究贡献到拓宽影响度衡量指标的范围。
在过去的几十年里,科学界已经从单独作者转向合著,这种转变显著且稳定,尤其在高引用作品中更为明显[18,129,130]。鉴于这种转变,一个研究领域——“团队科学的科学“(the science of team science)[131,132]已经出现,致力于研究促进或阻碍团队效率的机制。团队规模可以通过论文的合著者数量来代理,研究显示,这可以预测不同类型的进步:较大的团队倾向于发展思想,而较小的团队倾向于打破当前的思维方式[17]。团队特性可以从合著者的背景中推断[133,134,135],从而量化团队在领域、年龄、性别或种族方面的多样性。基于合著的合作网络[130,136,137,138,139]提供了微妙的基于网络的指标,以理解个人和机构的合作。
然而,仅仅使用合著关系来研究团队存在一些限制[132]。首先,合著关系可能掩盖了个体的角色[140,141,142],这促使机构采取措施来分配功劳,包括作者排序和个人贡献声明[56,143]。其次,合著关系不能反映团队成员之间复杂的动力学和相互作用,而这些往往对团队的成功至关重要[53,144]。第三,合作贡献可以超越出版物中的合著关系,包括研究实验室的成员或者共同研究负责人(co-PIs)在研究项目上的贡献[145,146]。像CRediT这样的倡议可能有助于解决其中一些问题,通过记录每个贡献者的详细角色[147]。
(三)机构
研究机构,如部门、大学、国家实验室和公司,涵盖了更广泛的研究人员及其相应的产出。机构成员资格可以从出版物或专利上列出的隶属关系推断出来[148,149],而机构的产出可以汇总所有隶属研究人员的成果[150]。机构研究信息系统(CRIS)包含了员工更全面的研究产出和活动。
一些研究问题考虑整个机构,调查研发投资的回报[104]、资源分配的不平等[22]和科学家的流动[21,148,149]。另一些研究问题关注机构的结构作为研究生产力的来源,深入了解同行效应的角色[125,151,152,153],机构政策如何影响研究结果[154,155],以及跨学科努力是否能促进创新[55]。以机构为导向的测量,面临着与个人和团队分析相似的限制,包括针对特定机构的名称消歧,以及正式出版记录对描述相关机构结果全貌的有限能力。如何在与一篇论文相关的多个机构之间分配学术声誉也是个难题。此外,相关机构的员工不仅限于发表研究的学者:实习生、技术人员和行政人员都对研究工作有所贡献[130]。
总之,测量使研究人员能够从多个维度量化科学产出和应用,但同时也提出了如何构造效度的问题:所提出的度量标准是否真正反映了想要衡量的内容?验证构造的效度非常重要,同时理解构造的局限性也同样关键。在可行的情况下,采用替代测量方法,或者运用访谈和调查等定性方法,可以提升测量的准确性和研究发现的稳健性。
四、经验方法
在本节中,我们回顾了两大类广泛的经验方法(见表1),每一类都有其独特的目标:(1)发现、估计和预测经验规律;(2)识别因果机制。对于每一种方法,我们提供一个具体示例以解释该方法是如何运作的,概括相关工作供感兴趣的读者参考,并探讨其贡献与局限性。
表1 科学学研究中的经验方法类别方法核心目标和贡献描述性和预测性方法经验规律和可推广的事实建立关于科学的观察规律;确认或否定现有的理论或假设;提供激发新理论的新发现 经典回归进行关于变量之间关系的正式假设检验,并估计其精确性和大小 机械模型模拟基本数据生成过程;提供可证伪的形式理论测试 机器学习用多个抽象层次表示数据;提供更高的预测准确性 因果方法匹配和固定效应减少回归估计中的偏差,更接近因果推断;为可能驱动相关性的特征提供更有效的控制 准实验利用数据背景中的固有随机性,允许对变量之间的因果推断 实验在良好控制的环境中构建正式实验,进行因果推断并研究特定的机制和干预措施
(一)描述性和预测性方法
1. 经验规律和可推广的事实
科学中经验规律的发现在推动概念发展和未来研究方向方面起着关键作用。通过观察大规模的经验模式,研究人员揭示了塑造科学的核心事实,并呈现了科学进展和实践理论必须解释的核心特征。例如,考虑引用分布。德·索拉·普赖斯(de Solla Price)首次提出引用分布的尾部较重[39],表明少数论文具有极高的引用量,而大多数论文引用量相对较少甚至没有引用。普赖斯提出“引用分布是幂律”,而研究人员后来改进了这一观点,表明该分布呈现对数正态分布,这是一个几乎普遍存在的规律,适用于不同时间和领域[156,157]。引用分布的尾部较重及其在科学界的普遍性反过来引发了大量理论工作,旨在解释这一关键的经验规律[20,156,158,159]。
经验规律通常令人惊讶,并且可能挑战先前对科学工作方式的信念。例如,已经证明,不同领域中伟大成就的年龄分布在中年时期达到顶峰[107,121,160],这与通常认为年轻科学家推动科学突破的普遍信念相悖。对个体职业的更深入研究还揭示,各个人之间的生产力模式存在广泛差异[29]。此外,学者的高影响力论文在其整个工作历程中呈现的频率显著稳定 [30,31]。
经验规律的发现在塑造关于科学本质的观点[10,45,161,162]、突破性思想的来源[15,163,164,165]、科学职业[21,29,126,127]、观点网络与科学家网络的结构[23,98,136,137,138,139,166]、性别不平等[57,108,126,135,143,167,168]以及科学家和科学机构关注的许多其他领域[22,47,86,97,102,105,134,169,170,171]中发挥了重要作用。同时,必须小心确保所发现的并不仅仅是由于数据选择或固有偏见产生的人为因素。为了区分虚假模式与有意义的模式,通过不同的筛选标准或非重叠数据源对发现进行强化测试非常关键。
2. 回归分析
在研究变量之间的相关性时,一种经典的方法是回归分析,它估计了一组变量如何解释感兴趣的结果的变异。回归分析可用来检验明确的假设或进行结果预测。例如,研究人员已经探讨了论文的新颖性是否能预测其被引用的影响[172]。通过在回归分析中添加额外的控制变量,可以进一步检验焦点关系的稳健性。
虽然回归分析在假设检验方面有用,但其确实存在重大限制。如果所提出的问题涉及“因果”关系,而非相关关系,回归分析则不适合这项任务,因为无法控制所有可能的混淆因素。未能考虑这些“遗漏变量”可能使回归系数估计偏差,从而导致错误的解释。此外,回归模型的拟合优度通常较低(R²值较小),这暗示着所考虑的变量对结果变化解释甚微。由于回归分析通常专注于以简单函数形式表现的特定关系,所以更强调可解释性而非整体预测能力。大规模数据集和新颖计算技术所推动的预测方法的出现为建模复杂关系带来了新机遇,具有更强的预测力。
3. 机制模型
机制建模是解释经验规律的重要方法,借鉴了主要用于物理学的方法。这种模型通过对基本元素之间的微观相互作用进行建模,使用可解释和可修改的公式来预测系统的宏观规律。虽然本质上是理论性的,但科学学中的机制模型通常以经验为基础,并且这种方法随着大规模、高分辨率数据的出现而发展起来。
简洁是机制模型的核心价值。例如,考虑为什么引用遵循重尾分布。普赖斯[159]将引用行为建模为一个在不断增长的引用网络上的累积优势过程,并发现如果一篇论文被引用的概率与其现有引用成线性增长,那么得到的分布将遵循幂律。这与实证观察大致一致。该模型有意简化、忽略了众多因素。然而,简单的累积优势过程本身足以解释引用的幂律分布。通过这种方式,机制模型可以帮助揭示能够解释观察到的模式的关键机制。
此外,随着经验证据的发展,机制模型可以得到改进。例如,后续研究表明引用分布更适合以对数正态分布来描述[156,173],促使研究人员引入适应性因素来概括论文吸引引用的固有差异[174,175]。此外,早期的论文被引用的概率不如预期[176,177,178],这促使了较新模型[20]引入了额外的老化效应[179]。通过结合累积优势、适应性和老化效应,我们已经可以在整个系统的总体特性以及个别论文的引用动力学方面取得相当大的预测能力[20]。
除了引用外,学界已经开发了机制模型来理解合作的形成[136,180,181,182,183]、知识的发现和传播[184,185]、主题选择[186,187]、职业动力学[30,31,188,189]、科学领域的增长[190]以及科学和其他领域中的失败动力学[178]。
同时,一些观察者认为机制模型过于简单,无法捕捉复杂现实世界问题的本质[191]。虽然它一直是自然科学的基石,但用一组有限的数学方程来表示社会现象可能会忽略使社会现象本身有趣的复杂性和异质性。这些担忧不仅限于科学学的领域,它们代表了计算社会科学中更广泛的主题[192,193],涵盖了社交网络[194,195]、人类流动性[196,197]和流行病[198,199]等领域。其他观察者对机制模型的实际效用以及它们是否能够用于指导决策和制定可行政策提出了质疑。尽管如此,存在上述限制,仍然可以通过简单的机制模型很好地捕捉到科学学中的一些复杂现象,展现复杂互动系统下的高度规律性,并提供有关科学本质的有力见解。将这种建模方法与其他方法相结合可能在未来的研究中特别有成果。
4. 机器学习
科学学的目标之一是预测科学研究的有潜力的方向。近年来,机器学习方法大大提高了预测能力[200,201],并在科学学中扮演着越来越重要的角色。与以往的方法相比,机器学习不强调假设或理论,而是依赖数据中的复杂关系并优化拟合好度来进行预测和分类。
传统的机器学习模型包括有监督学习、半监督学习和无监督学习。模型的选择取决于数据的可用性和研究问题,范围从用于引文预测的有监督模型[202,203]到用于社区检测的无监督模型[204]。以科学知识的映射为例[94,205,206]。无监督方法应用网络聚类算法来映射科学的结构。相关的可视化工具能够解读底层网络中的聚类,让观察者看到科学知识的组织、互动和演化。最近,监督学习,尤其是深度神经网络,经历了特别快速的发展[207]。神经网络可以对诸如图像和文本等非结构化数据生成高维表示,其中编码了人类专家难以识别的复杂属性。
以文本分析为例。最近的一项研究[52]利用了330万篇材料科学论文摘要,预测材料的热电性能。直观地说,用来描述材料的词语,可能预测其至今尚未发现的性能(图2)。与随机材料相比,该模型预测的材料在未来5年内被报道为热电材料的可能性增加了8倍,这表明机器学习有潜力大大加速知识发现,特别是随着数据的规模和范围不断增长。事实上,预测新发现的方向代表了机器学习模型最有前景的途径之一,神经网络广泛应用于生物学[208]、物理学[209,210]、数学[211]、化学[212]、医学[213]和临床应用[214]。神经网络还提供了一个定量框架,以探索从科学论文[53]、期刊[215]、组织[148],到绘画和电影[32]等创造性产品的特性。神经网络还可以帮助大规模预测各个学科论文的可重复性[53,216]。
图2 机器学习预测的一个例子。这个图示了word2vec skip-gram方法[52],目标是使用先前的科学文献预测材料的有用属性。a,word2vec skip-gram模型的架构和训练过程,三层全连接神经网络从文献中每个单词及其上下文的稀疏向量(输入层)中学习200维表示(隐藏层)。b,单词嵌入的前两个主成分。在二维空间中,具有相似特征的材料靠近,从而允许预测材料的属性。不同的目标单词以不同的颜色显示。
尽管机器学习能够提供高度准确的预测能力,但在科学学领域的成功应用面临着挑战,特别是在解释性方面。研究人员可能更看重透明和可解释的发现,以了解某个特征如何影响结果,而不是一个黑箱模型。缺乏解释性也引发了对偏见和公平性的担忧。在从数据中预测可重复模式时,机器学习模型不可避免地包含并复制了这些数据中嵌入的偏见,通常以不透明的方式呈现。机器学习在诸如刑事司法系统和招聘流程等方面的公平性问题备受争议[217]。因此,在科学学中有效而负责任地使用机器学习需要人与机器之间明智的合作,以构建一个可供审查和修改的可靠系统。
(二)因果方法
前述方法可以揭示科学运作的核心事实并发展预测能力。然而,它们无法捕捉因果关系,而这在评估政策干预方面尤为有用。例如,我们如何测试科学政策是促进还是阻碍个人、团队或机构的表现?因果方法的总体思想是构建某种反事实世界,在该世界中,两个群体除了一个群体经历了处理另一个群体没有经历的处理之外,其余方面完全相同。
1. 迈向因果关系
在进行因果方法之前,首先考虑观测数据的解释难题是很有助益的。由于观测数据来源于那些未完全了解或未能测量的作用机制,观察到的相关性可能由未在分析中涵盖的潜在因素驱动。这一挑战使得在观测数据中进行因果推断变得异常困难。意识到这个问题是解决它的第一步。这进一步促使了采用一些中介实证方法,包括使用匹配策略和固定效应,这些方法虽不能完全消除但可以帮助应对推断难题。我们首先考虑这些方法,然后再转向更全面的因果方法。
匹配。匹配通过利用丰富的信息,在处理组接受处理之前,构建一个在尽可能多的可观测特征上与处理组相似的对照组(未进行某种操作处理)。然后,可以通过比较处理组和匹配的对照组进行推断。精确匹配适用于分类值,例如国家、性别、学科或所属领域等[35,218]。粗糙精确匹配考虑连续变量的百分位区间,并在同一区间内匹配观测值[133]。倾向得分匹配则是根据控制变量估计接受“处理”的概率,并使用这些估计值来匹配处理组和对照组,从而将匹配任务从比较多个协变量的值简化为比较单个值[24,219]。对于随时间变化的变量,动态匹配非常有用[220,221]。
固定效应。固定效应是一种用于控制混杂因素的强有力工具,如今已成为标准方法[222,223,224]。使用固定效应的一个关键要求是对同一主体或实体(个人、领域、机构等)有多次观察。固定效应充当一个虚拟变量,体现了该实体的任何固定特性的作用。例如,有研究发现,性别多样化团队比同性别团队产生的论文影响力更高[225]。一个混杂因素可能是,倾向于撰写高影响力论文的个体,也更可能在性别多样化团队中工作。通过包括个体固定效应,可以考虑到可能驱动感兴趣关系的个体的任何固定特征(如智商、文化背景或先前教育)。
总之,匹配和固定效应方法可以减少解释变量之间关系时潜在的偏差来源。然而,在这些研究中可能仍存在混杂因素。例如,固定效应不能控制给定实体内随时间变化的未观察因素(例如,融资渠道或新技能的获取)。要令人信服地确定因果效应,通常需要采用不同的研究方法,我们接下来将转向这些方法。
2. 准实验
经济学和其他领域的研究人员已经开发出一系列准实验方法来构建处理组和对照组。这里的关键思想是利用外部事件的随机性,使受试者不同程度地接受特定的处理。在这里,我们回顾了三种准实验方法:双重差分法、工具变量法和回归不连续性(图3)。
图3 准实验方法。a–c,该图展示了(a)双重差分法、(b)工具变量和(c)回归不连续性方法的示意图。b中的实线表示因果关系,虚线表示在工具变量方法产生因果推断时不允许的关系。
双重差分法。双重差分法回归研究意外事件的影响,将受到某种操作影响的组(处理组)与未受影响的组(对照组)进行比较。对照组旨在提供反事实路径,即如果没有意外事件会发生什么。理想情况下,处理组和对照组在操作事件之前几乎处于相同的路径上,但如果组之间处于平行路径上,双重差分法也可以起作用(图3a)。例如,一篇论文[226]研究了明星科学家的过早死亡如何影响他们之前合作者的生产力。对照组是在时间范围内没有死亡的超级科学家的合作者。在死亡事件发生之前,两组在出版物方面没有显著差异,然而,在一位明星科学家去世后,处理组的合作者平均经历了与对照组相比5-8%的质量调整出版率下降。双重差分法在科学学领域具有广泛的适用性,已被用于分析资助设计的因果效应[24],以及先前研究的获取成本、大学技术转移政策、知识产权、引用实践、领域演化和论文撤稿的影响。双重差分法文献在经济学领域尤其迅速增长,并有大量最近的改进[233,234]。
工具变量。另一种准实验方法使用“工具变量”。目标是通过使用第三个工具变量来确定某个特征X对某个结果Y的因果影响。这个工具变量是一种准随机事件,它引起X的变化,并且除了通过X对结果Y产生影响外,对其他方面没有其他影响(图3b)。例如,一项关于天文学的研究,旨在了解望远镜使用时间如何影响科学家职业发展[235]。在这里,不能简单地看望远镜使用时间和职业结果之间的相关性,因为许多混淆因素(如才能或毅力)可能同时影响望远镜使用时间和职业机会。现在考虑天气作为一个工具变量。多云天气将随机减少天文学家的观测时间。然而,特定夜晚的天气不太可能与科学家的固有品质相关。天气可以提供一个工具变量来揭示望远镜使用时间和职业结果之间的因果关系。工具变量已被用于科学学研究中的本地同伴效应[151],科学委员会中性别构成的影响[236],对未来创新的专利 [237]和对发明者流动的税收政策[238]。
回归不连续性。在回归不连续性中,通过设置任意阈值来获得某些利益的政策可以构建处理组和对照组(图3c)。以拨款申请的资金拨款线为例。那些得分越接近拨款线的提案,在其可观察和不可观察特征上都越为相似,但只有那些得分超过拨款线的项目才能获得资金。例如,一项研究[110]探讨了赢得早期职业拨款对以后赢得中期职业拨款的概率的影响。该概率在初始拨款的拨款线上出现不连续跳跃,为估计获得拨款的因果效应提供了所需的处理组和对照组。该示例利用了“尖锐”的回归不连续性,即处理状态完全由截止点决定。如果我们假设处理状态仅部分由截止点决定,我们可以使用“模糊”的回归不连续性设计。在这里,获得拨款的概率用来估计未来的结果[11,110,239,240,241]。
虽然准实验是强大的工具,但它们也面临着自身的限制。首先,这些方法在特定背景下识别因果效应,通常涉及少量观察。对于更广泛的人群或背景,样本的代表性通常是一个悬而未决的问题。其次,因果设计的有效性通常不是十分牢固的。研究人员通常进行不同的鲁棒性检验,以验证处理前在处理组和对照组之间可观测混淆因素是否存在显著差异。然而,处理组和对照组之间可能仍存在不可观测的特征差异。工具变量的质量以及它们除了通过感兴趣的变量之外对结果没有影响的具体声明,也很难评。最终,研究人员必须在一定程度上依靠判断来确定是否满足因果推断的适当条件。
本节强调了流行的计量经济学方法来进行因果推断。其他实证方法,如基于图的因果建模[242,243],也代表了评估因果关系的重要研究方向。这些方法通常将因果关系表示为有向无环图,其中节点表示变量,箭头表示它们之间的因果联系。在科学学领域,有向无环图方法已被应用于量化期刊影响因子[244]和性别或种族偏见[245]对引用的因果效应。与计量经济学方法相比,因果图建模也引发了关于优势和劣势的讨论[246,247]。
3. 实验
与准实验方法相比,实验室和现场实验通过对处理和对照组进行直接随机分配。这些方法明确参与数据生成过程,通过操纵干预措施来观察反事实情况。这些实验旨在研究特定兴趣的机制,并通过设计实验和正式随机化,可以产生特别严格的因果推断。
实验室实验。实验室实验在良好控制的实验环境中构建对照世界。研究人员随机将参与者分配到处理组或对照组,然后操纵实验条件以观察两组之间的不同结果。例如,考虑关于团队表现和性别构成方面的实验室实验[144,248]。研究人员随机将参与者分配到不同的团队,进行解决难题或集思广益等任务。研究发现,女性比例较高的团队平均表现更好,这提供了性别多样性与团队表现之间因果关系的证据。实验室实验可以帮助研究人员测试其他难以观察到的力量,比如竞争如何影响创造力[249]。实验室实验还被用来评估期刊影响因子如何塑造科学家对奖励的看法[250],以及招聘中的性别偏见[251]。
实验室实验可以精确控制环境和程序,以隔离感兴趣的因果效应。然而,参与者在人造环境中的行为可能与现实世界中的环境不同,这引发了关于结果的普遍性和可复制性的问题[252,253,254]。为了评估现实世界中的因果效应,研究人员使用随机对照试验。
随机对照试验。随机对照试验或现场实验是各个学科中因果推断的基本方法。随机对照试验随机将参与者分配到处理组和对照组[255],并且不仅可以用于评估机制,还可以用于测试政策变化等真实世界的干预措施。科学的科学领域已经越来越多地使用随机对照试验。例如,一项现场实验[146]研究了降低合作伙伴搜索成本是否会增加在拨款申请中的合作。作者随机将主要研究员分配到医学院的面对面会议中,然后测量参与者共同撰写拨款提案的机会。随机对照试验还为同行评审[256,257,258,259,260]和科学中的性别偏见[261,262,263]提供了丰富的因果洞察。
虽然随机对照试验在科学学领域具有强大的能力,但由于两个主要原因,其难以进行。第一个原因涉及政策干预中的潜在风险。例如,尽管在个体之间随机分配资金能为资助者产生重要的因果洞察,但也可能意外地损害参与者的职业生涯[264]。第二,科学学中的关键问题通常需要长期追踪结果,这使得随机对照试验成本高昂。这也增加了复制研究结果的难度。早期讨论的准实验方法的一个相对优势是可以在历史记录中识别潜在长时间的因果效应。另一方面,准实验必须被发现而不是被设计,并且对于许多感兴趣的问题通常不可用。虽然最佳方法取决于具体情境,但越来越多的研究人员正在建立平台,以促进科学学领域的随机对照试验,旨在降低成本并扩大规模。与科学机构合作进行随机对照试验也可以为及时的、与政策相关的研究做出贡献,从而大幅度改善科学决策和投资。
五、展望
科学学研究得益于大规模数据的增长、新的测量手段和不断拓宽的实证方法领域。这些工具提供了巨大的能力,可以测试关于科学的概念框架,发现影响科学生产力的因素,预测关键的科学结果,并设计有助于更好促进未来科学进展的政策。对实证技术的仔细理解可以帮助研究人员选择感兴趣问题的有效工具,并推动科学学领域的发展。对这些方法论的更好和更广泛的理解,还可以在不同的研究社区之间建立桥梁,促进沟通和合作,并更好地利用多样化的观点的价值。科学学是关于将科学方法运用到科学本体的探讨。这项工作的成果,随着时间的推移,可以指导研究人员和研究机构在各个科学探究领域中取得更大的进展,增进对科学的探索和理解。
参考文献
1. Bush, V. Science–the Endless Frontier: A Report to the President on a Program for Postwar Scientific Research (National Science Foundation, 1990).2. Mokyr, J. The Gifts of Athena (Princeton Univ. Press, 2011).3. Jones, B. F. in Rebuilding the Post-Pandemic Economy (eds Kearney, M. S. & Ganz, A.) 272–310 (Aspen Institute Press, 2021).4. Wang, D. & Barabási, A.-L. The Science of Science (Cambridge Univ. Press, 2021).5. Fortunato, S. et al. Science of science. Science 359, eaao0185 (2018).6. Azoulay, P. et al. Toward a more scientific science. Science 361, 1194–1197 (2018).7. Clauset, A., Larremore, D. B. & Sinatra, R. Data-driven predictions in the science of science. Science 355, 477–480 (2017).8. Zeng, A. et al. The science of science: from the perspective of complex systems. Phys. Rep. 714, 1–73 (2017).9. Lin, Z., Yin. Y., Liu, L. & Wang, D. SciSciNet: a large-scale open data lake for the science of science research. Sci. Data, https://doi. org/10.1038/s41597-023-02198-9 (2023).10. Ahmadpoor, M. & Jones, B. F. The dual frontier: patented inventions and prior scientific advance. Science 357, 583–587 (2017).11. Azoulay, P., Graff Zivin, J. S., Li, D. & Sampat, B. N. Public R&D investments and private-sector patenting: evidence from NIH funding rules. Rev. Econ. Stud. 86, 117–152 (2019).12. Yin, Y., Dong, Y., Wang, K., Wang, D. & Jones, B. F. Public use and public funding of science. Nat. Hum. Behav. 6, 1344–1350 (2022).13. Merton, R. K. The Sociology of Science: Theoretical and Empirical Investigations (Univ. Chicago Press, 1973).14. Kuhn, T. The Structure of Scientific Revolutions (Princeton Univ. Press, 2021).15. Uzzi, B., Mukherjee, S., Stringer, M. & Jones, B. Atypical combinations and scientific impact. Science 342, 468–472 (2013). 16. Zuckerman, H. Scientific Elite: Nobel Laureates in the United States(Transaction Publishers, 1977).17. Wu, L., Wang, D. & Evans, J. A. Large teams develop and small teams disrupt science and technology. Nature 566, 378–382 (2019).18. Wuchty, S., Jones, B. F. & Uzzi, B. The increasing dominance of teams in production of knowledge. Science 316, 1036–1039 (2007).19. Foster, J. G., Rzhetsky, A. & Evans, J. A. Tradition and innovation in scientists’ research strategies. Am. Sociol. Rev. 80, 875–908 (2015).20. Wang, D., Song, C. & Barabási, A.-L. Quantifying long-term scientific impact. Science 342, 127–132 (2013).21. Clauset, A., Arbesman, S. & Larremore, D. B. Systematic inequality and hierarchy in faculty hiring networks. Sci. Adv. 1, e1400005 (2015).22. Ma, A., Mondragón, R. J. & Latora, V. Anatomy of funded research in science. Proc. Natl Acad. Sci. USA 112,14760–14765 (2015).23. Ma, Y. & Uzzi, B. Scientific prize network predicts who pushes the boundaries of science. Proc. Natl Acad. Sci. USA 115, 12608–12615 (2018).24. Azoulay, P., Graff Zivin, J. S. & Manso, G. Incentives and creativity: evidence from the academic life sciences. RAND J. Econ. 42, 527–554 (2011).25. Schor, S. & Karten, I. Statistical evaluation of medical journal manuscripts. JAMA 195, 1123–1128 (1966).26. Platt, J. R. Strong inference: certain systematic methods of scientific thinking may produce much more rapid progress than others. Science 146, 347–353 (1964).27. Ioannidis, J. P. Why most published research findings are false. PLoS Med. 2, e124 (2005).28. Simonton, D. K. Career landmarks in science: individual differences and interdisciplinary contrasts. Dev. Psychol. 27, 119 (1991).29. Way, S. F., Morgan, A. C., Clauset, A. & Larremore, D. B. The misleading narrative of the canonical faculty productivity trajectory. Proc. Natl Acad. Sci. USA 114, E9216–E9223 (2017).30. Sinatra, R., Wang, D., Deville, P., Song, C. & Barabási, A.-L. Quantifying the evolution of individual scientific impact. Science 354, aaf5239 (2016).31. Liu, L. et al. Hot streaks in artistic, cultural, and scientific careers. Nature 559, 396–399 (2018).32. Liu, L., Dehmamy, N., Chown, J., Giles, C. L. & Wang, D. Understanding the onset of hot streaks across artistic, cultural, and scientific careers. Nat. Commun. 12, 5392 (2021).33. Squazzoni, F. et al. Peer review and gender bias: a study on 145 scholarly journals. Sci. Adv. 7, eabd0299 (2021).34. Hofstra, B. et al. The diversity–innovation paradox in science. Proc. Natl Acad. Sci. USA 117, 9284–9291 (2020).35. Huang, J., Gates, A. J., Sinatra, R. & Barabási, A.-L. Historical comparison of gender inequality in scientific careers across countries and disciplines. Proc. Natl Acad. Sci. USA 117, 4609–4616 (2020).36. Gläser, J. & Laudel, G. Governing science: how science policy shapes research content. Eur. J. Sociol. 57, 117–168 (2016).37. Stephan, P. E. How Economics Shapes Science (Harvard Univ. Press, 2012).38. Garfield, E. & Sher, I. H. New factors in the evaluation of scientific literature through citation indexing. Am. Doc. 14, 195–201 (1963).39. de Solla Price, D. J. Networks of scientific papers. Science 149, 510–515 (1965).40. Etzkowitz, H., Kemelgor, C. & Uzzi, B. Athena Unbound: The Advancement of Women in Science and Technology (Cambridge Univ. Press, 2000).41. Simonton, D. K. Scientific Genius: A Psychology of Science (Cambridge Univ. Press, 1988).42. Khabsa, M. & Giles, C. L. The number of scholarly documents on the public web. PLoS ONE 9, e93949 (2014).43. Xia, F., Wang, W., Bekele, T. M. & Liu, H. Big scholarly data: a survey. IEEE Trans. Big Data 3, 18–35 (2017).44. Evans, J. A. & Foster, J. G. Metaknowledge. Science 331, 721–725 (2011).45. Milojević, S. Quantifying the cognitive extent of science. J. Informetr. 9, 962–973 (2015).46. Rzhetsky, A., Foster, J. G., Foster, I. T. & Evans, J. A. Choosing experiments to accelerate collective discovery. Proc. Natl Acad. Sci. USA 112, 14569–14574 (2015).47. Poncela-Casasnovas, J., Gerlach, M., Aguirre, N. & Amaral, L. A. Large-scale analysis of micro-level citation patterns reveals nuanced selection criteria. Nat. Hum. Behav. 3, 568–575 (2019).48. Hardwicke, T. E. et al. Data availability, reusability, and analytic reproducibility: evaluating the impact of a mandatory open data policy at the journal Cognition. R. Soc. Open Sci. 5, 180448 (2018).49. Nagaraj, A., Shears, E. & de Vaan, M. Improving data access democratizes and diversifies science. Proc. Natl Acad. Sci. USA 117, 23490–23498 (2020).50. Bravo, G., Grimaldo, F., López-Iñesta, E., Mehmani, B. & Squazzoni, F. The effect of publishing peer review reports on referee behavior in five scholarly journals. Nat. Commun. 10, 322 (2019).51. Tran, D. et al. An open review of open review: a critical analysis of the machine learning conference review process. Preprint at https://doi.org/10.48550/arXiv.2010.05137 (2020).52. Tshitoyan, V. et al. Unsupervised word embeddings capture latent knowledge from materials science literature. Nature 571, 95–98 (2019).53. Yang, Y., Wu, Y. & Uzzi, B. Estimating the deep replicability of scientific findings using human and artificial intelligence. Proc. Natl Acad. Sci. USA 117, 10762–10768 (2020).54. Mukherjee, S., Uzzi, B., Jones, B. & Stringer, M. A new method for identifying recombinations of existing knowledge associated with high‐impact innovation. J. Prod. Innov. Manage. 33, 224–236 (2016).55. Leahey, E., Beckman, C. M. & Stanko, T. L. Prominent but less productive: the impact of interdisciplinarity on scientists’ research. Adm. Sci. Q. 62, 105–139 (2017).56. Sauermann, H. & Haeussler, C. Authorship and contribution disclosures. Sci. Adv. 3, e1700404 (2017).57. Oliveira, D. F. M., Ma, Y., Woodruff, T. K. & Uzzi, B. Comparison of National Institutes of Health grant amounts to first-time male and female principal investigators. JAMA 321, 898–900 (2019).58. Yang, Y., Chawla, N. V. & Uzzi, B. A network’s gender composition and communication pattern predict women’s leadership success. Proc. Natl Acad. Sci. USA 116, 2033–2038 (2019).59. Way, S. F., Larremore, D. B. & Clauset, A. Gender, productivity, and prestige in computer science faculty hiring networks. In Proc. 25th International Conference on World Wide Web 1169–1179. (ACM 2016)60. Malmgren, R. D., Ottino, J. M. & Amaral, L. A. N. The role of mentorship in protege performance. Nature 465, 622–626 (2010).61. Ma, Y., Mukherjee, S. & Uzzi, B. Mentorship and protégé success in STEM fields. Proc. Natl Acad. Sci. USA 117, 14077–14083 (2020).62. Börner, K. et al. Skill discrepancies between research, education, and jobs reveal the critical need to supply soft skills for the data economy. Proc. Natl Acad. Sci. USA 115, 12630–12637 (2018).63. Biasi, B. & Ma, S. The Education-Innovation Gap (National Bureau of Economic Research Working papers, 2020).64. Bornmann, L. Do altmetrics point to the broader impact of research? An overview of benefits and disadvantages of altmetrics. J. Informetr. 8, 895–903 (2014).65. Cleary, E. G., Beierlein, J. M., Khanuja, N. S., McNamee, L. M. & Ledley, F. D. Contribution of NIH funding to new drug approvals 2010–2016. Proc. Natl Acad. Sci. USA 115, 2329–2334 (2018).66. Spector, J. M., Harrison, R. S. & Fishman, M. C. Fundamental science behind today’s important medicines. Sci. Transl. Med. 10, eaaq1787 (2018).67. Haunschild, R. & Bornmann, L. How many scientific papers are mentioned in policy-related documents? An empirical investigation using Web of Science and Altmetric data. Scientometrics 110, 1209–1216 (2017).68. Yin, Y., Gao, J., Jones, B. F. & Wang, D. Coevolution of policy and science during the pandemic. Science 371, 128–130 (2021).69. Sugimoto, C. R., Work, S., Larivière, V. & Haustein, S. Scholarly use of social media and altmetrics: a review of the literature. J. Assoc. Inf. Sci. Technol. 68, 2037–2062 (2017).70. Dunham, I. Human genes: time to follow the roads less traveled? PLoS Biol. 16, e3000034 (2018).71. Kustatscher, G. et al. Understudied proteins: opportunities and challenges for functional proteomics. Nat. Methods 19, 774–779 (2022).72. Rosenthal, R. The file drawer problem and tolerance for null results. Psychol. Bull. 86, 638 (1979).73. Franco, A., Malhotra, N. & Simonovits, G. Publication bias in the social sciences: unlocking the file drawer. Science 345, 1502–1505 (2014).74. Vera-Baceta, M.-A., Thelwall, M. & Kousha, K. Web of Science and Scopus language coverage. Scientometrics 121, 1803–1813 (2019).75. Waltman, L. A review of the literature on citation impact indicators. J. Informetr. 10, 365–391 (2016).76. Garfield, E. & Merton, R. K. Citation Indexing: Its Theory and Application in Science, Technology, and Humanities (Wiley, 1979).77. Kelly, B., Papanikolaou, D., Seru, A. & Taddy, M. Measuring Technological Innovation Over the Long Run Report No. 0898-2937 (National Bureau of Economic Research, 2018).78. Kogan, L., Papanikolaou, D., Seru, A. & Stoffman, N. Technological innovation, resource allocation, and growth. Q. J. Econ. 132, 665–712 (2017).79. Hall, B. H., Jaffe, A. & Trajtenberg, M. Market value and patent citations. RAND J. Econ. 36, 16–38 (2005).80. Yan, E. & Ding, Y. Applying centrality measures to impact analysis: a coauthorship network analysis. J. Am. Soc. Inf. Sci. Technol. 60, 2107–2118 (2009).81. Radicchi, F., Fortunato, S., Markines, B. & Vespignani, A. Diffusion of scientific credits and the ranking of scientists. Phys. Rev. E 80, 056103 (2009).82. Bollen, J., Rodriquez, M. A. & Van de Sompel, H. Journal status. Scientometrics 69, 669–687 (2006).83. Bergstrom, C. T., West, J. D. & Wiseman, M. A. The eigenfactorTM metrics. J. Neurosci. 28, 11433–11434 (2008).84. Cronin, B. & Sugimoto, C. R. Beyond Bibliometrics: Harnessing Multidimensional Indicators of Scholarly Impact (MIT Press, 2014).85. Hicks, D., Wouters, P., Waltman, L., De Rijcke, S. & Rafols, I. Bibliometrics: the Leiden Manifesto for research metrics. Nature 520, 429–431 (2015).86. Catalini, C., Lacetera, N. & Oettl, A. The incidence and role of negative citations in science. Proc. Natl Acad. Sci. USA 112, 13823–13826 (2015).87. Alcacer, J. & Gittelman, M. Patent citations as a measure of knowledge flows: the influence of examiner citations. Rev. Econ. Stat. 88, 774–779 (2006).88. Ding, Y. et al. Content‐based citation analysis: the next generation of citation analysis. J. Assoc. Inf. Sci. Technol. 65, 1820–1833 (2014).89. Teufel, S., Siddharthan, A. & Tidhar, D. Automatic classification of citation function. In Proc. 2006 Conference on Empirical Methods in Natural Language Processing, 103–110 (Association for Computational Linguistics 2006)90. Seeber, M., Cattaneo, M., Meoli, M. & Malighetti, P. Self-citations as strategic response to the use of metrics for career decisions. Res. Policy 48, 478–491 (2019).91. Pendlebury, D. A. The use and misuse of journal metrics and othercitationindicators.Arch.Immunol.Ther.Exp.57, 1–11 (2009).92. Biagioli, M. Watch out for cheats in citation game. Nature 535, 201 (2016).93. Jo, W. S., Liu, L. & Wang, D. See further upon the giants: quantifying intellectual lineage in science. Quant. Sci. Stud. 3, 319–330 (2022).94. Boyack, K. W., Klavans, R. & Börner, K. Mapping the backbone of science. Scientometrics 64, 351–374 (2005).95. Gates, A. J., Ke, Q., Varol, O. & Barabási, A.-L. Nature’s reach: narrowworkhasbroadimpact.Nature575,32–34(2019).96. Börner, K., Penumarthy, S., Meiss, M. & Ke, W. Mapping the diffusion of scholarly knowledge among major US research institutions. Scientometrics 68, 415–426 (2006).97. King, D. A. The scientific impact of nations. Nature 430, 311–316 (2004).98. Pan, R. K., Kaski, K. & Fortunato, S. World citation and collaboration networks: uncovering the role of geography in science. Sci. Rep. 2, 902 (2012).99. Jaffe, A. B., Trajtenberg, M. & Henderson, R. Geographic localization of knowledge spillovers as evidenced by patent citations. Q. J. Econ. 108, 577–598 (1993).100. Funk, R. J. & Owen-Smith, J. A dynamic network measure of technological change. Manage. Sci. 63, 791–817 (2017).101. Yegros-Yegros, A., Rafols, I. & D’este, P. Does interdisciplinary research lead to higher citation impact? The different effect of proximal and distal interdisciplinarity. PLoS ONE 10, e0135095 (2015).102. Larivière, V., Haustein, S. & Börner, K. Long-distance interdisciplinarity leads to higher scientific impact. PLoS ONE 10, e0122565 (2015).103. Fleming, L., Greene, H., Li, G., Marx, M. & Yao, D. Government-funded research increasingly fuels innovation. Science 364, 1139–1141 (2019).104. Bowen, A. & Casadevall, A. Increasing disparities between resource inputs and outcomes, as measured by certain health deliverables, in biomedical research. Proc. Natl Acad. Sci. USA 112, 11335–11340 (2015).105. Li, D., Azoulay, P. & Sampat, B. N. The applied value of public investments in biomedical research. Science 356, 78–81 (2017).106. Lehman, H. C. Age and Achievement (Princeton Univ. Press, 2017). 107. Simonton, D. K. Creative productivity: a predictive and explanatory model of career trajectories and landmarks. Psychol. Rev. 104, 66 (1997).108. Duch, J. et al. The possible role of resource requirements and academic career-choice risk on gender differences in publication rate and impact. PLoS ONE 7, e51332 (2012).109. Wang, Y., Jones, B. F. & Wang, D. Early-career setback and future career impact. Nat. Commun. 10, 4331 (2019).110. Bol, T., de Vaan, M. & van de Rijt, A. The Matthew effect in science funding. Proc. Natl Acad. Sci. USA 115, 4887–4890 (2018). 111. Jones, B. F. Age and great invention. Rev. Econ. Stat. 92, 1–14 (2010).112. Newman, M. Networks (Oxford Univ. Press, 2018).113. Mazloumian, A., Eom, Y.-H., Helbing, D., Lozano, S. & Fortunato, S. How citation boosts promote scientific paradigm shifts and nobel prizes. PLoS ONE 6, e18975 (2011).114. Hirsch, J. E. An index to quantify an individual’s scientific research output. Proc. Natl Acad. Sci. USA 102, 16569–16572 (2005).115. Alonso, S., Cabrerizo, F. J., Herrera-Viedma, E. & Herrera, F. h-index: a review focused in its variants, computation and standardization for different scientific fields. J. Informetr. 3, 273–289 (2009).116. Egghe, L. An improvement of the h-index: the g-index. ISSI Newsl. 2, 8–9 (2006).117. Kaur, J., Radicchi, F.&Menczer, F. Universality of scholarl yimpact metrics. J. Informetr. 7, 924–932 (2013).118. Majeti, D. et al. Scholar plot: design and evaluation of an information interface for faculty research performance. Front. Res. Metr. Anal. 4, 6 (2020).119. Sidiropoulos, A., Katsaros, D. & Manolopoulos, Y. Generalized Hirsch h-index for disclosing latent facts in citation networks. Scientometrics 72, 253–280 (2007).120. Jones, B. F. & Weinberg, B. A. Age dynamics in scientific creativity. Proc. Natl Acad. Sci. USA 108, 18910–18914 (2011).121. Dennis, W. Age and productivity among scientists. Science 123, 724–725 (156).122. Sanyal, D. K., Bhowmick, P. K. & Das, P. P. A review of author name disambiguation techniques for the PubMed bibliographic database. J. Inf. Sci. 47, 227–254 (2021).123. Haak, L. L., Fenner, M., Paglione, L., Pentz, E. & Ratner, H. ORCID: a system to uniquely identify researchers. Learn. Publ. 25, 259–264 (2012).124. Malmgren, R. D., Ottino, J. M. & Amaral, L. A. N. The role of mentorship in protégé performance. Nature 465, 662–667 (2010). 125. Oettl, A. Reconceptualizing stars: scientist helpfulness and peer performance. Manage. Sci. 58, 1122–1140 (2012).126. Morgan, A. C. et al. The unequal impact of parenthood in academia. Sci. Adv. 7, eabd1996 (2021).127. Morgan, A. C. et al. Socioeconomic roots of academic faculty. Nat. Hum. Behav. 6, 1625–1633 (2022).128. San Francisco Declaration on Research Assessment (DORA) (American Society for Cell Biology, 2012).129. Falk‐Krzesinski, H. J. et al. Advancing the science of team science. Clin. Transl. Sci. 3, 263–266 (2010).130. Cooke, N. J. et al. Enhancing the Effectiveness of Team Science (National Academies Press, 2015).131. Börner, K. et al. A multi-level systems perspective for the science of team science. Sci. Transl. Med. 2, 49cm24 (2010).132. Leahey, E. From sole investigator to team scientist: trends in the practice and study of research collaboration. Annu. Rev. Sociol. 42, 81–100 (2016).133. AlShebli, B. K., Rahwan, T. & Woon, W. L. The preeminence of ethnic diversity in scientific collaboration. Nat. Commun. 9, 5163 (2018).134. Hsiehchen, D., Espinoza, M. & Hsieh, A. Multinational teams and diseconomies of scale in collaborative research. Sci. Adv. 1, e1500211 (2015).135. Koning, R., Samila, S. & Ferguson, J.-P. Who do we invent for? Patentsbywomenfocusmoreonwomen’shealth,butfew women get to invent. Science 372, 1345–1348 (2021).136. Barabâsi, A.-L. et al. Evolution of the social network of scientific collaborations. Physica A 311, 590–614 (2002).137. Newman, M. E. Scientific collabo ration networks. I. Network construction and fundamental results. Phys. Rev. E 64, 016131 (2001).138. Newman, M. E. Scientific collaboration networks. II. Shortest paths, weighted networks, and centrality. Phys. Rev. E 64, 016132 (2001).139. Palla, G., Barabási, A.-L. & Vicsek, T. Quantifying social group evolution. Nature 446, 664–667 (2007).140. Ross, M. B. et al. Women are credited less in science than men. Nature 608, 135–145 (2022).141. Shen, H. -W.&Barabási, A. -L. Collective credital location in science. Proc. Natl Acad. Sci. USA 111, 12325–12330 (2014).142. Merton, R. K. Matthew effect in science. Science 159, 56–63 (1968).143. Ni, C., Smith, E., Yuan, H., Larivière, V. & Sugimoto, C. R. The gendered nature of author ship. Sci. Adv. 7, eabe4639(2021).144. Woolley, A. W., Chabris, C. F., Pentland, A., Hashmi, N. & Malone, T. W. Evidence for a collective intelligence factor in the performance of human groups. Science 330, 686–688 (2010).145. Feldon, D. F. et al. Postdocs’ lab engagement predicts trajectories of PhD students’ skill development. Proc. Natl Acad. Sci. USA 116, 20910–20916 (2019).146. Boudreau, K. J. et al. A field experiment on search costs and the formation of scientific collaborations. Rev. Econ. Stat. 99, 565–576 (2017).147. Holcombe, A. O. Contributorship, not author ship: use CRediT to indicate who did what. Publications 7, 48 (2019).148. Murray, D. et al. Unsupervised embedding of trajectories captures the latent structure of mobility. Preprint at https://doi. org/10.48550/arXiv.2012.02785 (2020).149. Deville, P. et al. Career on the move: geography, stratification, and scientific impact. Sci. Rep. 4, 4770 (2014).150. Edmunds, L. D. et al. Why do women choose or reject careers in academic medicine? A narrative review of empirical evidence. Lancet 388, 2948–2958 (2016).151. Waldinger, F. Peer effects in science: evidence from the dismissal of scientists in Nazi Germany. Rev. Econ. Stud. 79, 838–861 (2012).152. Agrawal, A., McHale, J. & Oettl, A. How stars matter: recruiting and peer effects in evolutionary biology. Res. Policy 46, 853–867 (2017).153. Fiore, S. M. Interdisciplinarity as teamwork: how the science of teams can inform team science. Small Group Res. 39, 251–277(2008).154. Hvide, H. K. & Jones, B. F. University innovation and the professor’s privilege. Am. Econ. Rev. 108, 1860–1898 (2018).155. Murray, F., Aghion, P., Dewatripont, M., Kolev, J. & Stern, S. Of mice and academics: examining the effect of openness on innovation. Am. Econ. J. Econ. Policy 8, 212–252 (2016).156. Radicchi, F., Fortunato, S. & Castellano, C. Universality of citation distributions: toward an objective measure of scientific impact. Proc. Natl Acad. Sci. USA 105, 17268–17272 (2008).157. Waltman, L., van Eck, N. J. & van Raan, A. F. Universality of citation distributions revisited. J. Am. Soc. Inf. Sci. Technol. 63, 72–77 (2012).158. Barabási, A.-L. & Albert, R. Emergence of scaling in random networks. Science 286, 509–512 (1999).159. de Solla Price, D. A general theory of bibliometric and other cumulative advantage processes. J. Am. Soc. Inf. Sci. 27, 292–306 (1976).160. Cole, S. Age and scientific performance. Am. J. Sociol. 84, 958–977 (1979).161. Ke, Q., Ferrara, E., Radicchi, F. & Flammini, A. Defining and identifying sleeping beauties in science. Proc. Natl Acad. Sci. USA 112, 7426–7431 (2015).162. Bornmann, L., de Moya Anegón, F. & Leydesdorff, L. Do scientific advancements lean on the shoulders of giants? A bibliometric investigation of the Ortega hypothesis. PLoS ONE 5, e13327 (2010).163. Mukherjee, S., Romero, D. M., Jones, B. & Uzzi, B. The nearly universal link between the age of past knowledge and tomorrow’s breakthroughs in science and technology: the hotspot. Sci. Adv. 3, e1601315 (2017).164. Packalen, M. & Bhattacharya, J. NIH funding and the pursuit of edge science. Proc. Natl Acad. Sci. USA 117, 12011–12016 (2020).165. Zeng, A., Fan, Y., Di, Z., Wang, Y. & Havlin, S. Fresh teams are associated with original and multidisciplinary research. Nat. Hum. Behav. 5, 1314–1322 (2021).166. Newman, M. E. The structure of scientific collaboration networks. Proc. Natl Acad. Sci. USA 98, 404–409 (2001).167. Larivière, V., Ni, C., Gingras, Y., Cronin, B. & Sugimoto, C. R. Bibliometrics: global gender disparities in science. Nature 504, 211–213 (2013).168. West, J. D., Jacquet, J., King, M. M., Correll, S. J. & Bergstrom, C. T. The role of gender in scholarly authorship. PLoS ONE 8, e66212 (2013).169. Gao, J., Yin, Y., Myers, K. R., Lakhani, K. R. & Wang, D. Potentially long-lasting effects of the pandemic on scientists. Nat. Commun. 12, 6188 (2021).170. Jones, B. F., Wuchty, S. & Uzzi, B. Multi-university research teams: shiftingimpact,geography,andstratificationinscience.Science 322, 1259–1262 (2008).171. Jones, B. F., Wuchty, S. & Uzzi, B. Multi-university research teams: shifting impact, geography, and stratification in science. Science 322, 1259–1262 (2008).172. Wang, J., Veugelers, R. & Stephan, P. Bias against novelty in science: a cautionary tale for users of bibliometric indicators. Res. Policy 46, 1416–1436 (2017).173. Stringer, M. J., Sales-Pardo, M. & Amaral, L. A. Statistical validation of a global model for the distribution of the ultimate number of citations accrued by papers published in a scientific journal. J.Assoc.Inf.Sci.Technol.61,1377–1385(2010).174. Bianconi, G. & Barabási, A.-L. Bose-Einstein condensation in complex networks. Phys. Rev. Lett. 86, 5632 (2001).175. Bianconi, G. & Barabási, A.-L. Competition and multiscaling in evolving networks. Europhys. Lett. 54, 436 (2001).176. Yin, Y. & Wang, D. The time dimension of science: connecting the past to the future. J. Informetr. 11, 608–621 (2017).177. Pan,R.K.,Petersen,A.M.,Pammolli,F.&Fortunato,S.The memory of science: Inflation, myopia, and the knowledge network. J. Informetr. 12, 656–678 (2018).178. Yin, Y., Wang, Y., Evans, J. A. & Wang, D. Quantifying the dynamics of failure across science, startups and security. Nature 575, 190–194 (2019).179. Candia, C. & Uzzi, B. Quantifying the selective forgetting and integration of ideas in science and technology. Am. Psychol. 76, 1067 (2021).180. Milojević, S. Principles of scientific research team formation and evolution. Proc. Natl Acad. Sci. USA 111, 3984–3989 (2014).181. Guimera, R., Uzzi, B., Spiro, J. & Amaral, L. A. N. Team assembly mechanisms determine collaboration network structure and team performance. Science 308, 697–702 (2005).182. Newman, M. E. Coauthorship networks and patterns of scientific collaboration. Proc. Natl Acad. Sci. USA 101, 5200–5205 (2004).183. Newman, M. E. Clustering and preferential attachment in growing networks. Phys. Rev. E 64, 025102 (2001).184. Iacopini, I., Milojević, S. & Latora, V. Network dynamics of innovation processes. Phys. Rev. Lett. 120, 048301 (2018).185. Kuhn, T., Perc, M. & Helbing, D. Inheritance patterns in citation networks reveal scientific memes. Phys. Rev. 4, 041036 (2014).186. Jia, T., Wang, D. & Szymanski, B. K. Quantifying patterns of research-interest evolution. Nat. Hum. Behav. 1, 0078 (2017).187. Zeng, A. et al. Increasing trend of scientists to switch between topics. Nat. Commun. https://doi.org/10.1038/s41467-019-11401-8 (2019).188. Siudem, G., Żogała-Siudem, B., Cena, A. & Gagolewski, M. Three dimensions of scientific impact. Proc. Natl Acad. Sci. USA 117, 13896–13900 (2020).189. Petersen, A. M. et al. Reputation and impact in academic careers. Proc. Natl Acad. Sci. USA 111, 15316–15321 (2014).190. Jin, C., Song, C., Bjelland, J., Canright, G. & Wang, D. Emergence of scaling in complex substitutive systems. Nat. Hum. Behav. 3, 837–846 (2019).191. Hofman, J. M. et al. Integrating explanation and prediction in computational social science. Nature 595, 181–188 (2021).192. Lazer, D. et al. Computational social science. Science 323, 721–723 (2009).193. Lazer, D. M. et al. Computational social science: obstacles and opportunities. Science 369, 1060–1062 (2020).194. Albert, R. & Barabási, A.-L. Statistical mechanics of complex networks. Rev. Mod. Phys. 74, 47 (2002).195. Newman, M. E. The structure and function of complex networks. SIAM Rev. 45, 167–256 (2003).196. Song, C., Qu, Z., Blumm, N. & Barabási, A.-L. Limits of predictability in human mobility. Science 327, 1018–1021 (2010).197. Alessandretti, L., Aslak, U. & Lehmann, S. The scales of human mobility. Nature 587, 402–407 (2020).198. Pastor-Satorras, R. & Vespignani, A. Epidemic spreading in scale-free networks. Phys. Rev. Lett. 86, 3200 (2001).199. Pastor-Satorras, R., Castellano, C., Van Mieghem, P. & Vespignani, A. Epidemic processes in complex networks. Rev. Mod. Phys. 87, 925 (2015).200.Goodfellow, I., Bengio, Y. & Courville, A. Deep Learning (MIT Press, 2016).201. Bishop, C. M. Pattern Recognition and Machine Learning (Springer, 2006).202. Dong, Y., Johnson, R. A. & Chawla, N. V. Will this paper increase your h-index? Scientific impact prediction. In Proc. 8th ACM International Conference on Web Search and Data Mining, 149–158 (ACM 2015)203.Xiao, S. et al. On modeling and predicting individual paper citation count over time. In IJCAI, 2676–2682 (IJCAI, 2016)204.Fortunato, S. Community detection in graphs. Phys. Rep. 486, 75–174 (2010).205. Chen, C. Science mapping: a systematic review of the literature. J. Data Inf. Sci. 2, 1–40 (2017).206.Van Eck, N. J. & Waltman, L. Citation-based clustering of publications using CitNetExplorer and VOSviewer. Scientometrics 111, 1053–1070 (2017).207. LeCun, Y., Bengio, Y. & Hinton, G. Deep learning. Nature 521, 436–444 (2015).208.Senior, A. W. et al. Improved protein structure prediction using potentials from deep learning. Nature 577, 706–710 (2020).209. Krenn, M. & Zeilinger, A. Predicting research trends with semantic and neural networks with an application in quantum physics. Proc. Natl Acad. Sci. USA 117, 1910–1916 (2020).210. Iten, R., Metger, T., Wilming, H., Del Rio, L. & Renner, R. Discovering physical concepts with neural networks. Phys. Rev. Lett. 124, 010508 (2020).211. Guimerà, R. et al. A Bayesian machine scientist to aid in the solution of challenging scientific problems. Sci. Adv. 6, eaav6971 (2020).212. Segler, M. H., Preuss, M. & Waller, M. P. Planning chemical syntheses with deep neural networks and symbolic AI. Nature 555, 604–610 (2018).213. Ryu, J. Y., Kim, H. U. & Lee, S. Y. Deep learning improves prediction of drug–drug and drug–food interactions. Proc. Natl Acad. Sci. USA 115, E4304–E4311 (2018).214. Kermany, D. S. et al. Identifying medical diagnoses and treatable diseases by image-based deep learning. Cell 172, 1122–1131.e9 (2018).215. Peng, H., Ke, Q., Budak, C., Romero, D. M. & Ahn, Y.-Y. Neural embeddings of scholarly periodicals reveal complex disciplinary organizations. Sci. Adv. 7, eabb9004 (2021).216. Youyou, W., Yang, Y. & Uzzi, B. A discipline-wide investigation of the replicability of psychology papers over the past two decades. Proc. Natl Acad. Sci. USA 120, e2208863120 (2023).217. Mehrabi, N., Morstatter, F., Saxena, N., Lerman, K. & Galstyan, A. A survey on bias and fairness in machine learning. ACM Computing Surveys (CSUR) 54, 1–35 (2021).218. Way, S. F., Morgan, A. C., Larremore, D. B. & Clauset, A. Productivity, prominence, and the effects of academic environment. Proc. Natl Acad. Sci. USA 116, 10729–10733 (2019).219. Li, W., Aste, T., Caccioli, F. & Livan, G. Early coauthorship with top scientists predicts success in academic careers. Nat. Commun. 10, 5170 (2019).220. Hendry, D. F., Pagan, A. R. & Sargan, J. D. Dynamic specification. Handb. Econ. 2, 1023–1100 (1984).221. Jin, C., Ma, Y. & Uzzi, B. Scientific prizes and the extraordinary growth of scientific topics. Nat. Commun. 12, 5619 (2021).222. Azoulay, P., Ganguli, I. & Zivin, J. G. The mobility of elite life scientists: professional and personal determinants. Res. Policy 46, 573–590 (2017).223. Slavova, K., Fosfuri, A. & De Castro, J. O. Learning by hiring: the effects of scientists’ inbound mobility on research performance in academia. Organ. Sci. 27, 72–89 (2016).224. Sarsons, H. Recognition for group work: gender differences in academia. Am. Econ. Rev. 107, 141–145 (2017).225. Campbell, L. G., Mehtani, S., Dozier, M. E. & Rinehart, J. Gender-heterogeneous working groups produce higher quality science. PLoS ONE 8, e79147 (2013).226. Azoulay, P., Graff Zivin, J. S. & Wang, J. Superstar extinction. Q. J. Econ. 125, 549–589 (2010).227. Furman, J. L. & Stern, S. Climbing atop the shoulders of giants: the impact of institutions on cumulative research. Am. Econ. Rev. 101, 1933–1963 (2011).228. Williams, H. L. Intellectual property rights and innovation: evidence from the human genome. J. Polit. Econ. 121, 1–27 (2013).229. Rubin, A. & Rubin, E. Systematic Bias in the Progress of Research. J. Polit. Econ. 129, 2666–2719 (2021).230. Lu, S. F., Jin, G. Z., Uzzi, B. & Jones, B. The retraction penalty: evidence from the Web of Science. Sci. Rep. 3, 3146 (2013).231. Jin, G. Z., Jones, B., Lu, S. F. & Uzzi, B. The reverse Matthew effect: consequences of retraction in scientific teams. Rev. Econ. Stat. 101, 492–506 (2019).232. Azoulay, P., Bonatti, A. & Krieger, J. L. The career effects of scandal: evidence from scientific retractions. Res. Policy 46, 1552–1569 (2017).233. Goodman-Bacon, A. Difference-in-differences with variation in treatment timing. J. Econ. 225, 254–277 (2021).234. Callaway, B. & Sant’Anna, P. H. Difference-in-differences with multiple time periods. J. Econ. 225, 200–230 (2021).235. Hill, R. Searching for Superstars: Research Risk and Talent Discovery in Astronomy Working Paper (Massachusetts Institute of Technology, 2019).236. Bagues, M., Sylos-Labini, M. & Zinovyeva, N. Does the gender composition of scientific committees matter? Am. Econ. Rev. 107, 1207–1238 (2017).237. Sampat, B. & Williams, H. L. How do patents affect follow-on innovation? Evidence from the human genome. Am. Econ. Rev. 109, 203–236 (2019).238. Moretti, E. & Wilson, D. J. The effect of state taxes on the geographical location of top earners: evidence from star scientists. Am. Econ. Rev. 107, 1858–1903 (2017).239. Jacob, B. A. & Lefgren, L. The impact of research grant funding on scientific productivity. J. Public Econ. 95, 1168–1177 (2011).240. Li, D. Expertise versus bias in evaluation: evidence from the NIH. Am. Econ. J. Appl. Econ. 9, 60–92 (2017).241. Wang, Y., Jones, B. F. & Wang, D. Early-career setback and future career impact. Nat. Commun. 10, 4331 (2019).242. Pearl, J. Causal diagrams for empirical research. Biometrika 82, 669–688 (1995).243. Pearl, J. & Mackenzie, D. The Book of Why: The New Science of Cause and Effect (Basic Books, 2018).244. Traag, V. A. Inferring the causal effect of journals on citations. Quant. Sci. Stud. 2, 496–504 (2021).245. Traag, V. & Waltman, L. Causal foundations of bias, disparity and fairness. Preprint at https://doi.org/10.48550/arXiv.2207.13665 (2022).246. Imbens, G. W. Potential outcome and directed acyclic graph approaches to causality: relevance for empirical practice in economics. J. Econ. Lit. 58, 1129–1179 (2020).247. Heckman, J. J. & Pinto, R. Causality and Econometrics (National Bureau of Economic Research, 2022).248. Aggarwal, I., Woolley, A. W., Chabris, C. F. & Malone, T. W. The impact of cognitive style diversity on implicit learning in teams. Front. Psychol. 10, 112 (2019).249. Balietti, S., Goldstone, R. L. & Helbing, D. Peer review and competition in the Art Exhibition Game. Proc. Natl Acad. Sci. USA 113, 8414–8419 (2016).250. Paulus, F. M., Rademacher, L., Schäfer, T. A. J., Müller-Pinzler, L. & Krach, S. Journal impact factor shapes scientists’ reward signal in the prospect of publication. PLoS ONE 10, e0142537 (2015).251. Williams, W. M. & Ceci, S. J. National hiring experiments reveal 2:1 faculty preference for women on STEM tenure track. Proc. Natl Acad. Sci. USA 112, 5360–5365 (2015).252. Collaboration, O. S. Estimating the reproducibility of psychological science. Science 349, aac4716 (2015).253. Camerer, C. F. et al. Evaluating replicability of laboratory experiments in economics. Science 351, 1433–1436 (2016).254. Camerer, C. F. et al. Evaluating the replicability of social science experiments in Nature and Science between 2010 and 2015. Nat. Hum. Behav. 2, 637–644 (2018).255. Duflo, E. & Banerjee, A. Handbook of Field Experiments (Elsevier, 2017).256. Tomkins, A., Zhang, M. & Heavlin, W. D. Reviewer bias in single versus double-blind peer review. Proc. Natl Acad. Sci. USA 114, 12708–12713 (2017).257. Blank, R. M. The effects of double-blind versus single-blind reviewing: experimental evidence from the American Economic Review. Am. Econ. Rev. 81, 1041–1067 (1991).258. Boudreau, K. J., Guinan, E. C., Lakhani, K. R. & Riedl, C. Looking across and looking beyond the knowledge frontier: intellectual distance, novelty, and resource allocation in science. Manage. Sci. 62, 2765–2783 (2016).259. Lane, J. et al. When Do Experts Listen to Other Experts? The Role of Negative Information in Expert Evaluations for Novel Projects Working Paper #21-007 (Harvard Business School, 2020).260. Teplitskiy, M. et al. Do Experts Listen to Other Experts? Field Experimental Evidence from Scientific Peer Review (Harvard Business School, 2019).261. Moss-Racusin, C. A., Dovidio, J. F., Brescoll, V. L., Graham, M. J. & Handelsman, J. Science faculty’s subtle gender biases favor male students. Proc. Natl Acad. Sci. USA 109, 16474–16479 (2012).262. Forscher, P. S., Cox, W. T., Brauer, M. & Devine, P. G. Little race or gender bias in an experiment of initial review of NIH R01 grant proposals. Nat. Hum. Behav. 3, 257–264 (2019).263. Dennehy, T. C. & Dasgupta, N. Female peer mentors early in college increase women’s positive academic experiences and retention in engineering. Proc. Natl Acad. Sci. USA 114, 5964–5969 (2017).264. Azoulay, P. Turn the scientific method on ourselves. Nature 484, 31–32 (2012).
(参考文献可上下滑动查看)
计算社会科学读书会第二季
计算社会科学作为一个新兴交叉领域,越来越多地在应对新冠疫情、舆论传播、社会治理、城市发展、组织管理等社会问题和社科议题中发挥作用,大大丰富了我们对社会经济复杂系统的理解。相比于传统社会科学研究,计算社会科学广泛采用了计算范式和复杂系统视角,因而与计算机仿真、大数据、人工智能、统计物理等领域的前沿方法密切结合。为了进一步梳理计算社会科学中的各类模型方法,推动研究创新,集智俱乐部发起了计算社会科学系列读书会。
【计算社会科学读书会】第二季由清华大学罗家德教授领衔,卡内基梅隆大学、密歇根大学、清华大学、匹兹堡大学的多位博士生联合发起,进行了12周的分享和讨论,一次闭门茶话会,两次圆桌讨论。本季读书聚焦讨论Graph、Embedding、NLP、Modeling、Data collection等方法及其与社会科学问题的结合,并针对性讨论预测性与解释性、人类移动、新冠疫情、科学学研究等课题。欢迎从事相关研究或对计算社会科学感兴趣的朋友参与学习。
详情请见:
推荐阅读
1. 推动跨学科研究,什么样的科研资助更有效?2. 大语言模型做科研的N种可能性:从自主进行科学实验到写综述文章3. 引文网络分析揭示全球科研不平等加剧:混圈子正阻止新想法诞生4. 张江:第三代人工智能技术基础——从可微分编程到因果推理 | 集智学园全新课程5. 成为集智VIP,解锁全站课程/读书会6. 加入集智,一起复杂!
点击“阅读原文”,报名读书会
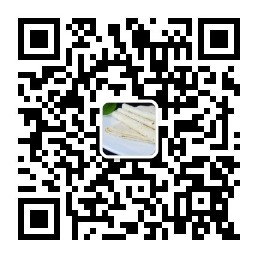