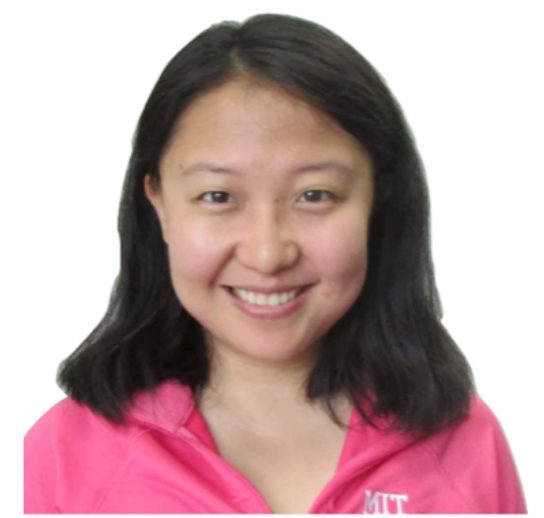
分享嘉宾杨梦娇
杨梦娇是Google DeepMind的高级研究科学家。她的研究兴趣包括模仿学习、深度强化学习(RL)、顺序决策、和基础模型。她的工作涵盖了离线强化学习、顺序决策的表示学习,以及控制、规划和RL的生成建模。她在2022年和2023年的NeurIPS发起了“决策基础模型”的学习会,将视觉、语言、规划和RL的研究社区聚集在一起,以大规模解决复杂的决策制定任务。杨梦娇在MIT获得了学士和硕士学位,由 Patrick Winston 和 Julian Shun 指导。
Sherry is a senior research scientist at Google DeepMind. Her research interests include imitation learning, deep reinforcement learning (RL), and recently foundation models for decision making. Her work spans offline reinforcement learning, representation learning for sequential decision making, and generative modeling for control, planning, and RL. She initiated the Foundation Models for Decision Making workshop at NeurIPS 2022 and 2023, bringing together research communities in vision, language, planning, and RL to solve complex decision making tasks at scale. Before her current role, Sherry received her Bachelor’s and Master’s degree from MIT advised by Patrick Winston and Julian Shun.
分享主题:基础模型与顺序决策的问题、方法、及应用
基础模型在互联网数据的训练下可以获取广泛的知识。随着这些模型的持续发展,它们不可避免地面临超越其数据驱动行为的挑战,尤其是在面对需要扩展推理、搜索或优化的复杂任务的低数据情境下。这样的任务一直是顺序决策的核心,涵盖了规划和强化学习等领域。顺序决策传统上面临着样本效率和泛化的挑战,部分原因是因为无法将来自互联网数据的广泛知识纳入其中。
在这次讲座中,我将提供三种受基础模型启发的方法,包括表示学习、生成建模和重新利用大型视觉和语言模型,以便利用来自基础模型的广泛知识来解决更为复杂的任务,如自动化控制、无人驾驶、机器人操纵和游戏等。
Foundation models pretrained on internet vision and language data have acquired broad knowledge. As these models continue to evolve, they inevitably face the challenge of outperforming their data-driven behaviors especially in low-data situations when faced with intricate tasks demanding extended reasoning, search, or optimization. Such tasks have been at the core of sequential decision making, encompassing areas such as planning and reinforcement learning. Sequential decision making has traditionally faced the challenges of sample efficiency and generalization, partially due to the inability to incorporate broad knowledge from internet data. In this talk, I will provide three foundation model inspired approaches including representation learning, conditional generative modeling, and repurposing pretrained vision and language models, in order to leverage broad knowledge from foundation models to solve more complex tasks such as continuous control, navigation, robotic manipulation, and game play.
活动时间:11月02日(周四)11:00-12:00
活动形式:线上直播,扫描下方二维码报名
线上交流:点击原文链接,还可有更多互动
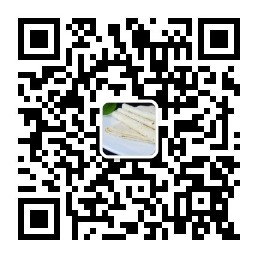