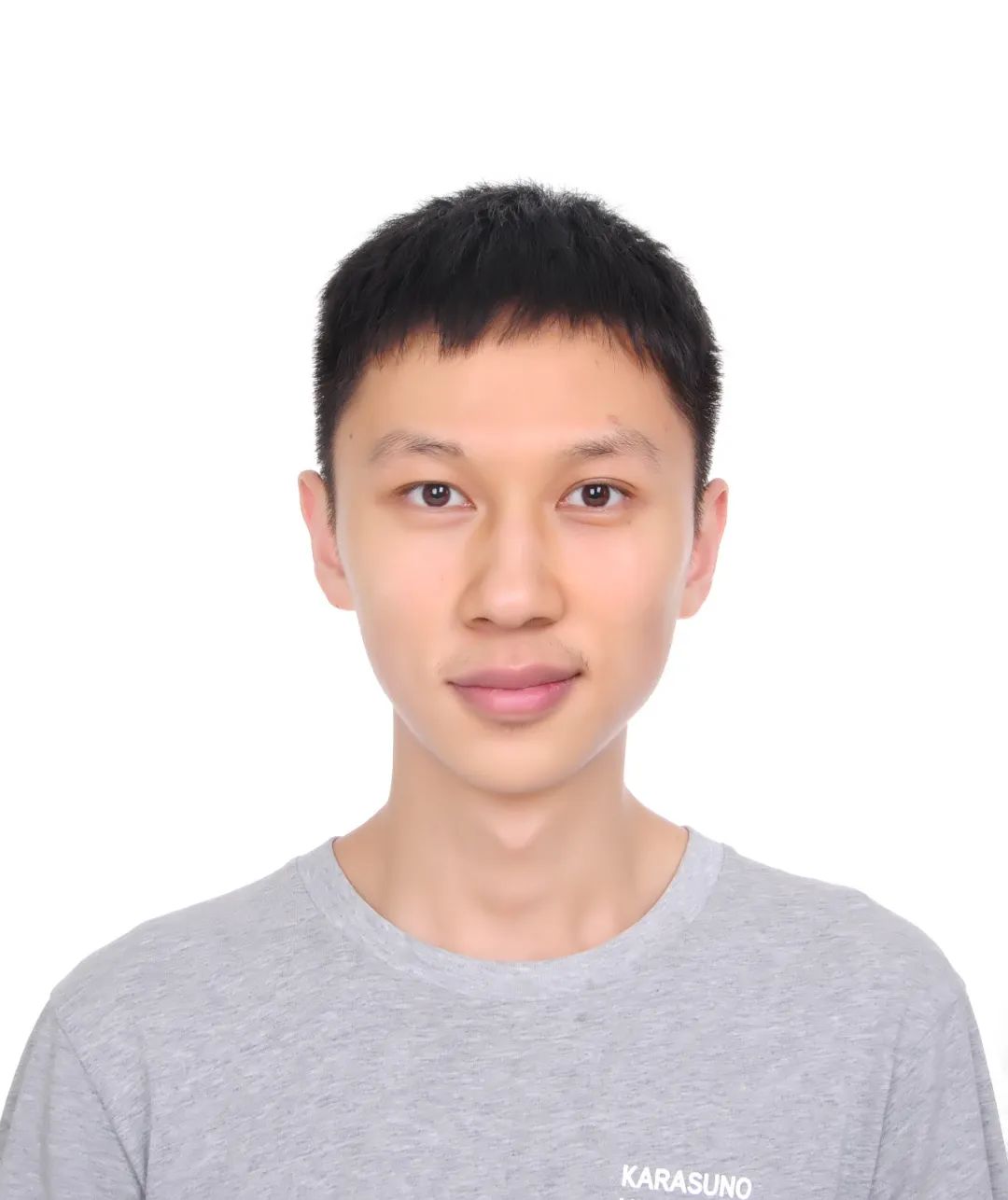
王宇飞
王宇飞是卡内基梅隆大学机器人研究所三年级博士生。他由 Zackory Erickson 教授和 David Held 教授共同指导。他于 2020 年 12 月在 David Held 教授的指导下获得卡内基梅隆大学计算机科学系计算机科学硕士学位。
在来到卡耐基梅隆大学之前,他于2019 年 7 月获得北京大学元培学院数据科学学士学位,导师为董彬教授。他的主要研究兴趣是机器人学习。他的研究生学习得到了优步总统奖学金的支持。
Yufei Wang is a third year Phd student at Robotics Institute, Carnegie Mellon University. He is co-advised by Prof. Zackory Erickson and Prof. David Held. He received M.S. in Computer Science from Computer Science Department, Carnegie Mellon University in Dec, 2020, advised by Prof. David Held.
Before coming to CMU, he received B.S. in Data Science from Yuanpei College, Peking University in July 2019, advised by Prof. Bin Dong. His general research interest is robot learning. His graduate study is supported by the Uber Presidential Fellowship.
RoboGen:通过生成模拟释放无限数据来实现自动化机器人学习
报告将围绕RoboGen进行介绍,RoboGen是一种生成式的机器人智能体,可以通过“生成模拟”自动大规模学习各种机器人技能。
RoboGen 利用基础模型和生成模型的最新进展。我们不直接使用或调整这些模型来产生策略或低级动作,而是提倡一种生成方案,该方案使用这些模型自动生成多样化的任务、场景和训练监督,从而在最少的人类监督下扩大机器人技能的学习。
我们的方法为机器人智能体配备了一个自我引导的提议-生成-学习循环:智能体首先提出要开发的有趣任务和技能,然后通过使用适当的空间配置填充相关对象和资产来生成相应的模拟环境。然后,智能体将提议的高级任务分解为子任务,选择最佳学习方法(强化学习、运动规划或轨迹优化),生成所需的训练监督,然后学习策略以获得提议的技能。
我们的工作试图提取大型模型中嵌入的广泛且通用的知识,并将其转移到机器人领域。我们的完全生成管道可以重复查询,产生与不同任务和环境相关的无穷无尽的技能演示。
Foundation models pretrained on internet vision and language data have acquired broad knowledge. As these models continue to evolve, they inevitably face the challenge of outperforming their data-driven behaviors especially in low-data situations when faced with intricate tasks demanding extended reasoning, search, or optimization. Such tasks have been at the core of sequential decision making, encompassing areas such as planning and reinforcement learning. Sequential decision making has traditionally faced the challenges of sample efficiency and generalization, partially due to the inability to incorporate broad knowledge from internet data.
In this talk, I will provide three foundation model inspired approaches including representation learning, conditional generative modeling, and repurposing pretrained vision and language models, in order to leverage broad knowledge from foundation models to solve more complex tasks such as continuous control, navigation, robotic manipulation, and game play.
活动时间:11月16日(周四)11:00-12:00
活动形式:线上直播,扫描下方二维码报名
线上交流:点击“阅读原文”,在智源社区一对一交流
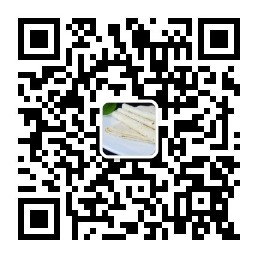