认识和理解视觉内容,以及基于人类指令对视觉世界进行推理,长久以来一直是一个具有挑战性问题。
最近,得益于大规模预训练,OpenAI 的 GPT-4V 展示了在自然语言处理任务和复杂视觉理解中令人印象深刻的能力。在12月20日,智源社区邀请了LLaVA-Plus相关作者来进行分享《视觉指令微调及工具使用构建多模态智能体》。
在这次演讲中,讲者将介绍 LLaVA,第一个在图像理解和推理方面具有类似 GPT-4V 级别的能力的开源项目,从而证明了这种方法可以以较低成本构建可定制的多模态大模型。
首先,介绍创建如何利用大语言模型,不需要大量手动注释的情况下,创建多模态指令微调数据集;并且这个方法成本可控,利用现有的预训练的大语言模型和视觉编码器,无需从头开始训练。此外,讲者将展示 LLaVA-1.5,仅通过对原始 LLaVA 进行简单修改,LLaVA-1.5 在 11 个基准测试中取得了SoTA。LLaVA-1.5 使用全公开数据集,一天内在单个 8-A100 节点上完成训练,并超过了包括Qwen-VL-Chat(使用十亿级数据)在内的方法。最后,讲者将展示一些 LLaVA 有趣的能力和限制,并概述我们渴望探索的方向。
提出了LLaVA-Plus,使用插件(视觉工具)提升多模态大语言模型的视觉能力。我们扩展了多模态大语言模型,使其支持了包括检测、分割、检索、生成、编辑在内的多种视觉能力。
LLaVA-Plus 维护着一个技能库,其中包含各种视觉和视觉语言预训练模型(工具),并且能够根据用户的多模式输入激活相关工具,以即时组合执行结果来完成许多现实任务。我们通过实验验证了LLaVA-Plus的有效性,在多个基准测试中取得了持续改进的结果,特别是在VisIT-Bench上达到了的新SoTA。论文地址:https://arxiv.org/pdf/2311.05437v1.pdf
Recognizing and understanding visual content, as well as reasoning about the visual world based on human instructions, has long been a challenging problem. Recently, OpenAI GPT-4V has showcased impressive capabilities in both NLP tasks and complex visual understanding challenges, thanks to large-scale pretraining and extensive instruction tuning. In this talk, I will introduce LLaVA, the first open-sourced project to demonstrate GPT-4V level capabilities in image understanding and reasoning. We demonstrate that this approach offers a promising path for building customizable, large multimodal models that follow human intent at an affordable cost. First, I will introduce how we approach this by creating a multimodal instruction-following dataset without the need for extensive manual annotations and by leveraging the existing pretrained LLMs and large vision encoders without the need of training-from-scratch. Additionally, I will present LLaVA-1.5, where it achieves SoTA on 11 benchmarks, with just simple modifications to the original LLaVA. It utilizes all public data, completes training in ~1 day on a single 8-A100 node, and surpasses methods like Qwen-VL-Chat that use billion-scale data. Finally, I will present some intriguing capabilities and limitations of LLaVA and outline a few future directions that we are eager to explore.
刘世隆,清华大学计算机系博士生。主要研究方向包括目标检测和分割,以及视觉-语言的多模态学习,尤其关心开放场景下的模型。
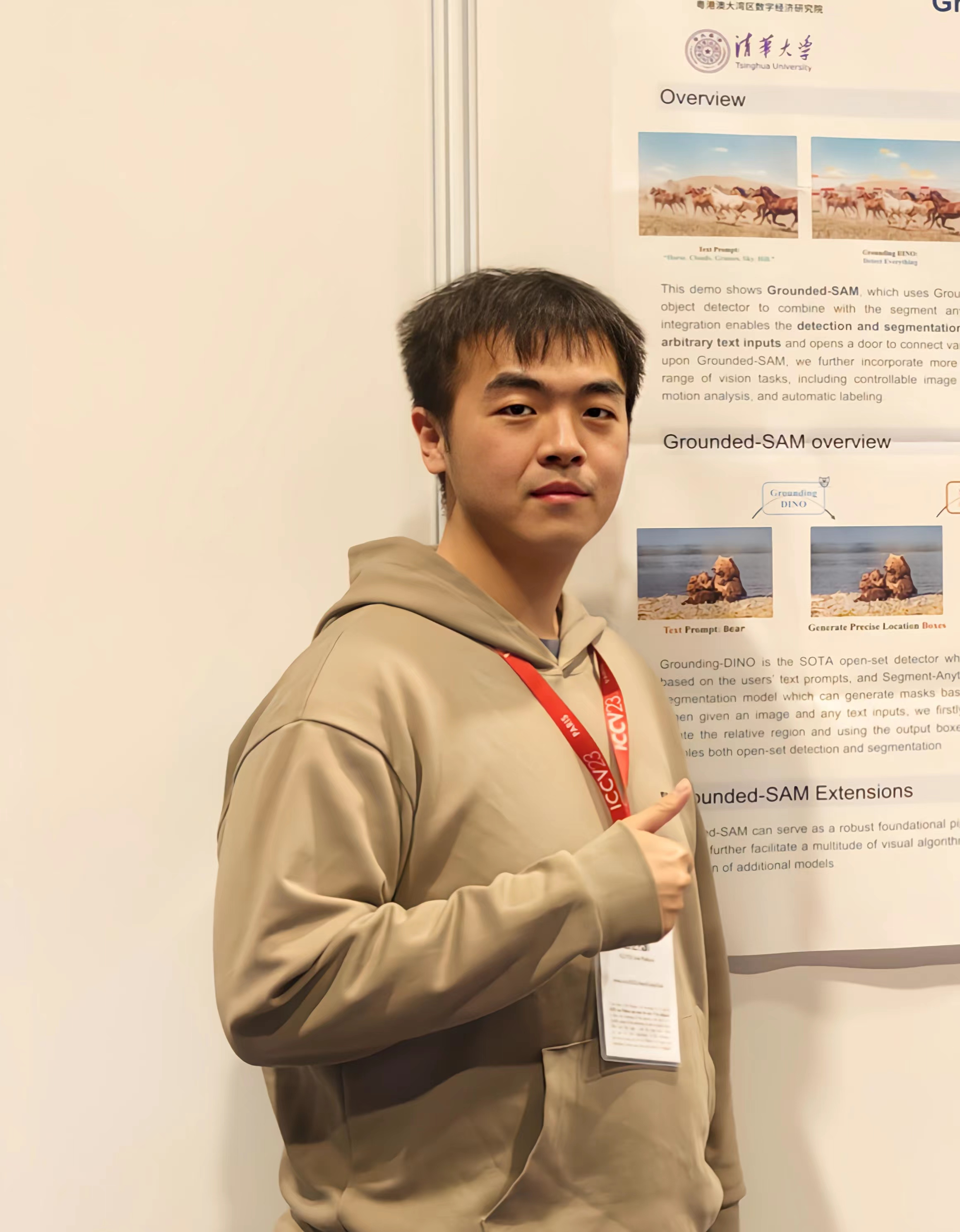
柳昊天,威斯康星麦迪逊分校计算机系博士生。最近的研究兴趣包括构建与对齐可定制的多模态大模型。
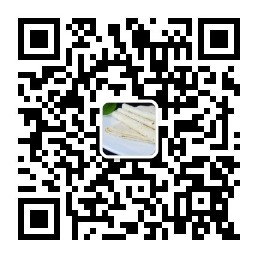