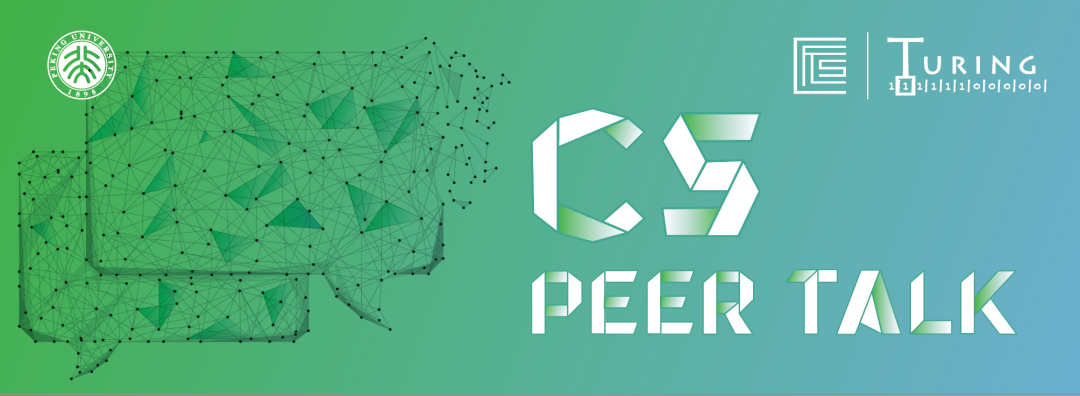
第三十九期
报告人:Weiqiang Zheng, Yale University
时间:1月13日(星期六)4:00pm
地点:静园五院204
报告信息
Title
Last-Iterate Convergence for Learning in Markov Games with Bandit Feedback
Abstract
Online learning in multi-player games captures many modern machine learning applications, ranging from generative adversarial networks and adversarial training to robust optimization and multi-agent reinforcement learning. Understanding last-iterate convergence in games is crucial since the last iterate characterizes the stability of the learning process and is widely used in practice.
In this talk, we study the problem of learning in two-player zero-sum Markov games, focusing on developing decentralized learning algorithms with non-asymptotic last-iterate convergence rates to Nash equilibrium. We first present a simple algorithm with last-iterate convergence rate in two-player zero-sum matrix games with bandit feedback. To the best of our knowledge, this is the first result that obtains finite last-iterate convergence rate given access to only bandit feedback. We then extend our result to the setting of two-player zero-sum Markov games, providing the first set of decentralized algorithms with non-asymptotic last-iterate/path convergence rates. This talk is based on joint work with Yang Cai, Haipeng Luo, and Chen-Yu Wei.
Biography
Weiqiang Zheng is a third-year PhD student in Computer Science at Yale University, advised by Prof. Yang Cai. He received his bachelor’s degree in Computer Science from Turing Class at Peking University. He has a broad research interest in game theory, online learning, and optimization.
about CS Peer Talk
作为活动的发起人,我们来自北京大学图灵班科研活动委员会,主要由图灵班各年级同学组成。我们希望搭建一个CS同学交流的平台,促进同学间的交流合作,帮助同学练习展示,同时增进友谊。
目前在计划中的系列包括但不限于:
-
教程系列:学生讲者为主,介绍自己的研究领域
-
研究系列:学生讲者为主,介绍自己的研究成果
-
客座系列:邀请老师做主题报告
除非报告人特别要求,报告默认是非公开的,希望营造一个自由放松但又互相激励的交流氛围。
主讲嘉宾招募
如果你愿意和大家分享你的学术成果、经历经验,总结回顾、触发新思,欢迎报名自荐。
主讲人报名:发邮件至 cs_research_tc@163.com,写明想讲的题目、内容及时间。
北京大学图灵班科研活动委员会
— 版权声明 —
本微信公众号所有内容,由北京大学前沿计算研究中心微信自身创作、收集的文字、图片和音视频资料,版权属北京大学前沿计算研究中心微信所有;从公开渠道收集、整理及授权转载的文字、图片和音视频资料,版权属原作者。本公众号内容原作者如不愿意在本号刊登内容,请及时通知本号,予以删除。
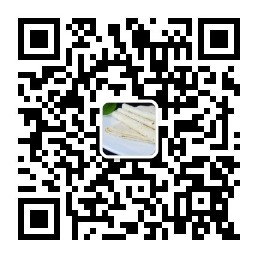