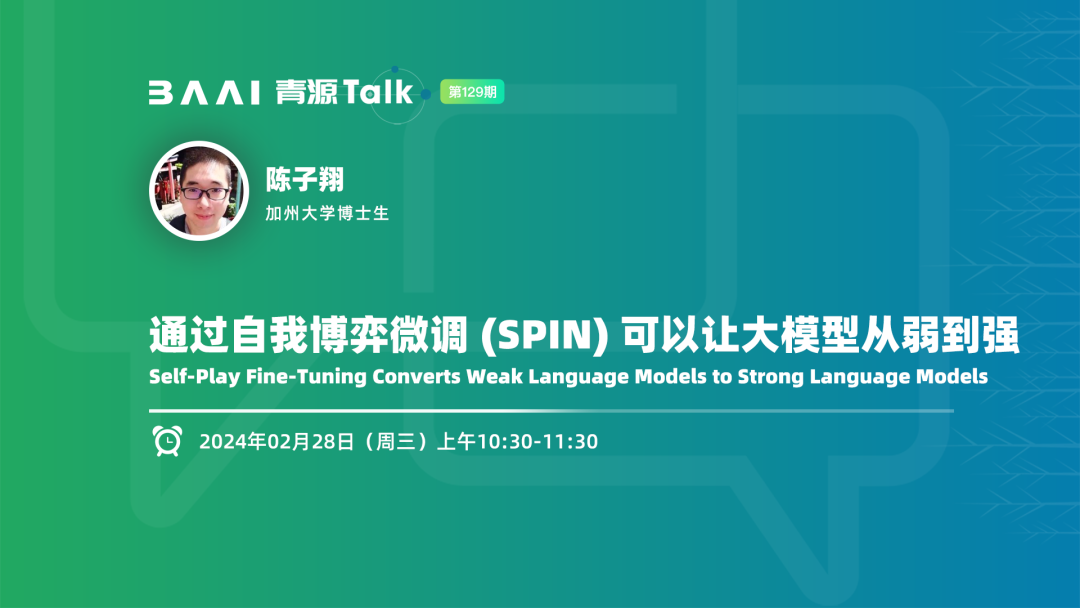
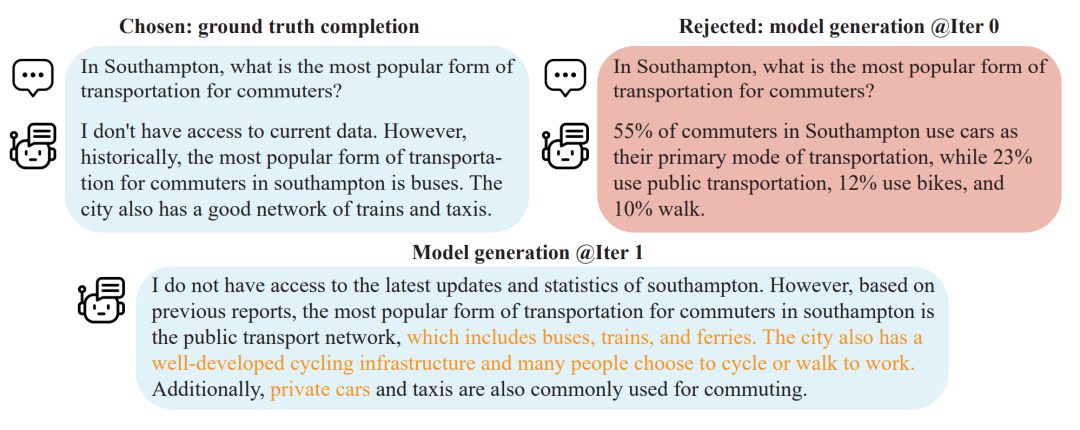
大模型的一大关键进步是训练之后的对齐过程,这能让模型的行为更符合需求。经典的对齐方法包括基于人类演示的监督式微调(SFT)和基于人类偏好反馈的强化学习(RLHF)。但这个过程却往往依赖于成本高昂的人类标注数据。因此,为了精简对齐过程,研究人员希望开发出能有效利用人类数据的微调方法。在这次演讲中,我将介绍我们最新的微调方法,自我博弈微调 (SPIN),它能在不需要额外标注数据的情况下进一步让LLMs自我改进。SPIN利用了一种自我博弈机制,通过与自身的实例进行交互从而提升其能力。具体来说,LLM从其之前的版本中生成训练数据,通过辨别这些自我生成的数据以及真实的标注数据,来自我提升。SPIN由此可以进一步发挥已标注数据的潜力。我们的实验结果显示,SPIN能在各种基准测试中提高LLM的性能,甚至能超越通过直接偏好优化(DPO)并补充额外的GPT-4 偏好数据训练的模型。另外,我还将概述我们方法的理论结果。更多详情以及我们的代码,可以访问我们的GitHub(https://github.com/uclaml/SPIN)
Harnessing the power of human-annotated data through Supervised Fine-Tuning (SFT) is pivotal for advancing Large Language Models (LLMs). In this talk, I will introduce our newest fine-tuning method, Self-Play Fine-Tuning (SPIN), which improves LLMs without the need for additional human-annotated data. SPIN utilizes a self-play mechanism, where the LLM enhances its capabilities by generating its own training data through interactions with instances of itself. Specifically, the LLM generates its own training data from its previous iterations, refining its policy by discerning these self-generated responses from those obtained from human-annotated data. As a result, SPIN unlocks the full potential of human-annotated data for SFT. Our empirical results show that SPIN can improve the LLM’s performance across a variety of benchmarks and even outperform models trained through direct preference optimization (DPO) supplemented with extra GPT-4 preference data. Additionally, I will outline the theoretical guarantees of our method. For more details and access to our codes, visit our GitHub repository (https://github.com/uclaml/SPIN).
报告嘉宾:陈子翔,加州大学洛杉矶分校(UCLA)计算机科学系的计算机科学博士生,导师是顾全全教授。他从清华大学获得了数学学士学位。他广泛地对深度学习、优化和控制的理论及其应用感兴趣,特别是在生成模型、特征学习和多智能体强化学习方面。最近他在研究利用生成模型在公共健康领域的科学应用。他曾是simons 计算理论研究所强化学习项目的访问研究生。扫描下方二维码
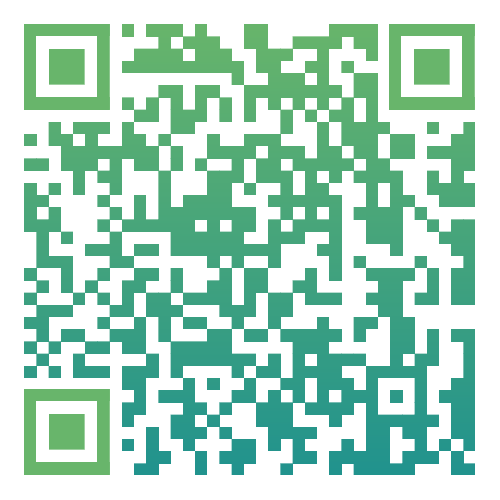
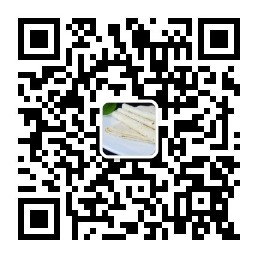