## 前言
我手头存了不少小说txt文档,一直都想整理一番,但是手工整理太累了所以也没动手。这半年来感觉我的python水平有了很大提升,于是打算写个程序帮我整理。
首先是去重,因为txt文档来源于不同地方,标题作者名什么的有可能不一致,内容上排版稍微差一点hash值也就变了,所以无论是靠文件名还是hash值检测重复文档都不可靠,干脆直接检测文本的相似度吧。网上搜了一下别人写的代码,整合了一下实现了一个最简单的文本相似度检测。
因为之前没接触过自然语言学习,所以本文的理解和代码可能有误,欢迎评论区指正。
(https://github.com/caly5144/shu-s-project/blob/master/text/)
## 原理
可以参考这两篇文章:
[https://blog.csdn.net/qq_43350003/article/details/105392702](https://blog.csdn.net/qq_43350003/article/details/105392702)
[https://www.cnblogs.com/airnew/p/9563703.html](https://www.cnblogs.com/airnew/p/9563703.html)
首先介绍一下什么是**词袋模型**。
例句:
* Jane wants to go to Shenzhen.
* Bob wants to go to Shanghai.
将所有词语装进一个袋子里,**不考虑其词法和语序的问题** ,即每个词语都是独立的。例如上面 2 个例句,就可以构成一个词袋,袋子里包括 Jane、wants、to、go、Shenzhen、Bob、Shanghai。假设建立一个数组(或词典)用于映射匹配
> [Jane, wants, to, go, Shenzhen, Bob, Shanghai]
那么上面两个例句就可以用以下两个向量表示,对应的下标与映射数组的下标相匹配,其值为该词语出现的次数
> [1,1,2,1,1,0,0]
> [0,1,2,1,0,1,1]
这两个词频向量就是词袋模型。
我们知道,一篇文章除了词频外,语序也是很重要的,词向量模型就是考虑**词语位置关系**的一种模型。因为这次任务并不是专业的查抄袭,所以词袋模型就足够了。
有了词频向量后,还需要一种指标来衡量两端文本间的相似度。本文采用**余弦相似度算法**,所谓余弦相似度算法,就是用一个向量空间中两个向量夹角间的余弦值作为衡量两个个体之间差异的大小,余弦值接近 1,夹角趋于 0,表明两个向量越相似,余弦值接近于 0,夹角趋于 90 度,表明两个向量越不相似。
公式如下:
$$
cos (theta)=frac{sum_{i=1}^{n}left(x_{i} times y_{i}right)}{sqrt{sum_{i=1}^{n}left(x_{i}right)^{2}} times sqrt{sum_{i=1}^{n}left(y_{i}right)^{2}}}
$$
## 代码
主要参考的就是这篇文章:[https://www.cnblogs.com/airnew/p/9563703.html](https://www.cnblogs.com/airnew/p/9563703.html)
我主要是将其numpy化,加快了运行速度。另外,考虑到txt文档的编码方式可能不一样,所以写了一个函数判断所打开文档的编码。
代码实现思路和上一节类似,首先用jieba分词将文档进行分词,然后去掉标点符号。为了防止介词状语等影响相似度的准确性,所以分词后要将这类词语去掉。本文使用了百度停用词,存放在`./stopwords`文件夹下,里面还有中文停用词表、哈工大停用词表、四川大学机器智能实验室停用词库,数据来源于[https://github.com/goto456/stopwords](https://github.com/goto456/stopwords),可自行修改。
接着就是生成两个文档的one-hot向量,计算出余弦相似度。代码如下:
“`python
import jieba
import re
import os
import numpy as np
import codecs
import chardet
class SimilarTest(object):
def __init__(self, a_path, b_path):
s1_code = self.get_code(a_path)
s2_code = self.get_code(b_path)
chars_pattern = re.compile(
r'[s·’!”#$%&'()#!()*+,-./:;<=>?@,:?¥★*、….>【】[]《》?“”‘’[]^_`{|}~。—]+’)
s1 = open(a_path, ‘r’, encoding=s1_code, errors=’ignore’).read()
s2 = open(b_path, ‘r’, encoding=s2_code, errors=’ignore’).read()
# 去除标点符号
self.s1 = chars_pattern.sub(“”, s1)
self.s2 = chars_pattern.sub(“”, s2)
# 设置停用词
self.stopwords = open(‘./stopwords/baidu_stopwords.txt’,
‘r’, encoding=’utf-8-sig’).read().splitlines()
def test(self):
s1_split = np.array(jieba.lcut(self.s1))
s2_split = np.array(jieba.lcut(self.s2))
s1_cut = s1_split[~np.isin(s1_split, self.stopwords)]
s2_cut = s2_split[~np.isin(s2_split, self.stopwords)]
word_set = np.union1d(s1_cut, s2_cut)
# 用字典保存两篇文章中出现的所有词并编上号
word_dict = dict()
for i, word in enumerate(word_set):
word_dict[word] = i
# 根据词袋模型统计词在每篇文档中出现的次数,形成向量
s1_cut_code = np.zeros(len(word_dict))
s2_cut_code = np.zeros(len(word_dict))
for word in s1_cut:
s1_cut_code[word_dict[word]] += 1
for word in s2_cut:
s2_cut_code[word_dict[word]] += 1
# 计算余弦相似度
try:
cos_arr = np.sum(s1_cut_code * s2_cut_code)
sq1 = np.power(s1_cut_code, 2).sum()
sq2 = np.power(s2_cut_code, 2).sum()
result = np.around(cos_arr / (np.sqrt(sq1) * np.sqrt(sq2)), 3)
except ZeroDivisionError:
result = 0.0
print(“文本相似度为:{}”.format(result))
@staticmethod
def get_code(path):
with open(path, ‘rb’) as file:
data = file.read(200)
dicts = chardet.detect(data)
return dicts[“encoding”]
if __name__ == ‘__main__’:
a_path = ‘atext.txt’
b_path = ‘btext.txt’
textST = SimilarTest(a_path, b_path)
textST.test()
“`
效果:
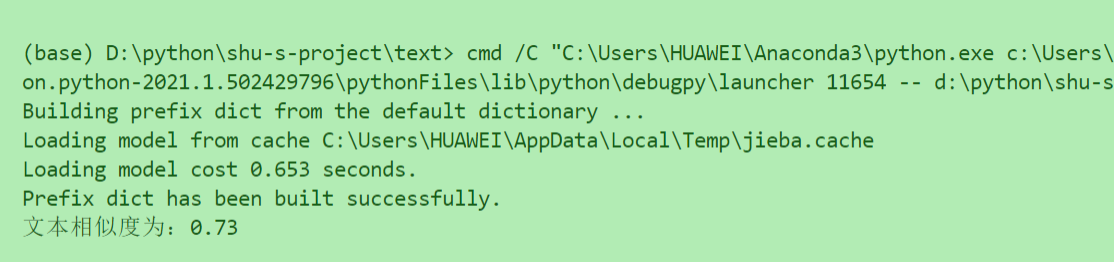Read More
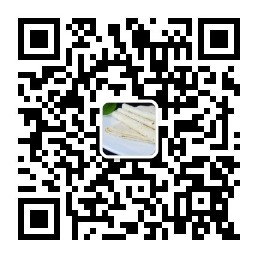