1、[LG] The Flan Collection: Designing Data and Methods for Effective Instruction Tuning
2、[CV] Attend-and-Excite: Attention-Based Semantic Guidance for Text-to-Image Diffusion Models
3、[LG] Simplex Random Features
4、[CL] Grounding Language Models to Images for Multimodal Generation
5、[LG] Mathematical Capabilities of ChatGPT
[CL] Faithful Chain-of-Thought Reasoning
CL] ThoughtSource: A central hub for large language model reasoning data
[LG] Scaling laws for single-agent reinforcement learning
[CL] LongEval: Guidelines for Human Evaluation of Faithfulness in Long-form Summarization
摘要:面向有效指令微调的数据和方法设计、基于注意力的文本到图像扩散模型语义指导、Simplex随机特征、面向多模态生成的语言模型到图像Grounding、ChatGPT的数学能力、忠实思维链推理、大型语言模型推理数据集成中心、单智能体强化学习的缩放律、长式摘要忠实度人工评估指南
1、[LG] The Flan Collection: Designing Data and Methods for Effective Instruction Tuning
S Longpre, L Hou, T Vu, A Webson, H W Chung, Y Tay, D Zhou, Q V. Le, B Zoph, J Wei, A Roberts
[Google Research]
The Flan Collection: 面向有效指令微调的数据和方法设计
要点:
一句话总结:
Flan-T5是一种公开可用的指令微调方法,通过用混合提示设置和其他关键技术(如任务平衡和丰富性)来提高性能。
摘要:
本文研究了公开可用的指令微调方法的设计决策,并对Flan 2022的方法进行了拆解。通过对Flan系列任务和方法的仔细消融研究,将设计决策的效果区分开来,这使得Flan-T5在不同的评估环境下比之前的工作要好3-17%以上。任务平衡和丰富性技术被忽视了,但对有效的指令微调至关重要,特别是在混合提示设置下的训练(零样本、少样本及思维链)实际上在所有设置中都能产生更强的性能(2%以上)。进一步的实验显示,Flan-T5需要更少的微调,可在单一的下游任务上比T5更高更快地收敛,使指令微调模型成为新任务的更计算高效的起点。
We study the design decisions of publicly available instruction tuning methods, and break down the development of Flan 2022 (Chung et al., 2022). Through careful ablation studies on the Flan Collection of tasks and methods, we tease apart the effect of design decisions which enable Flan-T5 to outperform prior work by 3-17%+ across evaluation settings. We find task balancing and enrichment techniques are overlooked but critical to effective instruction tuning, and in particular, training with mixed prompt settings (zero-shot, few-shot, and chain-of-thought) actually yields stronger (2%+) performance in all settings. In further experiments, we show Flan-T5 requires less finetuning to converge higher and faster than T5 on single downstream tasks, motivating instruction-tuned models as more computationally-efficient starting checkpoints for new tasks. Finally, to accelerate research on instruction tuning, we make the Flan 2022 collection of datasets, templates, and methods publicly available at this https URL.
https://arxiv.org/abs/2301.13688
2、[CV] Attend-and-Excite: Attention-Based Semantic Guidance for Text-to-Image Diffusion Models
H Chefer, Y Alaluf, Y Vinker, L Wolf, D Cohen-Or
[Tel-Aviv University]
Attend-and-Excite: 基于注意力的文本到图像扩散模型语义指导
要点:
-
提出生成语义护理(GSN)的概念,以减少文本到图像扩散模型中属性的灾难性忽视和不正确绑定; -
提出 Attend-and-Excite,一种基于注意力的 GSN 形式,旨在指导模型注意文本中的所有主题,并提高生成图像的忠实性; -
讨论 GSN 在文本条件生成以外的其他图像编辑和生成任务中的潜在应用。
一句话总结:
Attend-and-Excite 是一种基于注意力的语义指导方法,通过加强输入文本提示中所有主题 Token 的激活,提高了文本到图像传播模型的忠实性。
摘要:
最近的文本-图像生成模型在目标文本提示的指导下,显示了无与伦比的生成多样化和创造性图像的能力。虽然是革命性的,但目前最先进的扩散模型仍然可能无法生成完全传达给定文本提示语义的图像。本文分析了公开可用的 Stable Diffusion 模型,并评估了灾难性忽视的存在,即该模型未能从输入提示中生成一个或多个主题。此外,本文发现在某些情况下,该模型也不能正确地将属性(如颜色)与相应的主题绑定。为了帮助减轻这些失败的情况,本文提出了生成语义护理(GSN)的概念,试图在推理时对生成过程进行即时干预,以提高生成图像的忠实度。用一种基于注意力的 GSN 表述,称为”注意和激发”,引导模型完善交叉注意力单元,以注意文本提示中的所有主题标记,并加强——或激发——它们的激活,鼓励模型生成文本提示中描述的所有主题。本文将所提出方法与其他方法进行比较,证明它在一系列的文本提示中更忠实地传达了所需的概念。
Recent text-to-image generative models have demonstrated an unparalleled ability to generate diverse and creative imagery guided by a target text prompt. While revolutionary, current state-of-the-art diffusion models may still fail in generating images that fully convey the semantics in the given text prompt. We analyze the publicly available Stable Diffusion model and assess the existence of catastrophic neglect, where the model fails to generate one or more of the subjects from the input prompt. Moreover, we find that in some cases the model also fails to correctly bind attributes (e.g., colors) to their corresponding subjects. To help mitigate these failure cases, we introduce the concept of Generative Semantic Nursing (GSN), where we seek to intervene in the generative process on the fly during inference time to improve the faithfulness of the generated images. Using an attention-based formulation of GSN, dubbed Attend-and-Excite, we guide the model to refine the cross-attention units to attend to all subject tokens in the text prompt and strengthen – or excite – their activations, encouraging the model to generate all subjects described in the text prompt. We compare our approach to alternative approaches and demonstrate that it conveys the desired concepts more faithfully across a range of text prompts.
https://arxiv.org/abs/2301.13826
3、[LG] Simplex Random Features
I Reid, K Choromanski, V Likhosherstov, A Weller
[University of Cambridge & Google]
Simplex随机特征
要点:
-
提出简单随机特征(SimRF),一种对高斯和 softmax 核进行无偏近似的新机制; -
与正交随机特征(ORF)相比,证明了核估计器的均方误差(MSE)更低,从而在非参数分类和可扩展的 Transformer 训练中表现更好; -
提出独立于权重的 SimRF 和独立于权重的 SimRFs+ 变体,其中 SimRF 被证明是最佳的独立于权重的几何耦合正随机特征机制。
一句话总结:
提出Simplex随机特征(SimRF),一种新的随机特征机制,其均方误差低于正交随机特征。
摘要:
本文提出 Simplex 随机特征(SimRF),一种新的随机特征(RF)机制,通过随机投影向量的几何关联对 softmax 和高斯核进行无偏近似。本文证明 SimRF 在与权重无关的几何耦合正随机特征(PRF)机制中,为这些核的无偏估计提供了最小的均方误差(MSE),大大超过了之前最准确的正交随机特征,而且没有可观察到的额外成本。本文提出一个计算成本更高的 SimRFs+ 变体,证明它在更广泛的依赖权重的几何耦合方案(允许随机矢量方向和范数之间的相关性)族中是渐进式最优。广泛的实证研究,显示了 SimRF 在包括点核估计、非参数分类和可扩展 Transformer 等设置中提供的一致收益。
We present Simplex Random Features (SimRFs), a new random feature (RF) mechanism for unbiased approximation of the softmax and Gaussian kernels by geometrical correlation of random projection vectors. We prove that SimRFs provide the smallest possible mean square error (MSE) on unbiased estimates of these kernels among the class of weight-independent geometrically-coupled positive random feature (PRF) mechanisms, substantially outperforming the previously most accurate Orthogonal Random Features at no observable extra cost. We present a more computationally expensive SimRFs+ variant, which we prove is asymptotically optimal in the broader family of weight-dependent geometrical coupling schemes (which permit correlations between random vector directions and norms). In extensive empirical studies, we show consistent gains provided by SimRFs in settings including pointwise kernel estimation, nonparametric classification and scalable Transformers.
https://arxiv.org/abs/2301.13856
4、[CL] Grounding Language Models to Images for Multimodal Generation
J Y Koh, R Salakhutdinov, D Fried
[CMU]
面向多模态生成的语言模型到图像Grounding
要点:
-
提出多模态数据冻结检索自回归生成(FROMAGe)模型,通过图像描述和对比学习的视觉 grounding 大规模语言模型进行高效训练; -
证明自回归大规模语言模型可以在对输入文本更敏感的情况下执行文本到图像检索; -
表明预训练的纯文本大规模语言模型的现有功能可以用于视觉 grounded 任务。
一句话总结:
提出一种通过图像描述和对比学习的视觉 grounding 大规模语言模型进行高效训练,使它们能够处理和产生任意交错的图像和文本数据。FROMAGe 模型能产生连贯的多模态输出,并在各种任务中显示出强大的零样本性能。
摘要:
本文提出一种有效的方法,将预训练的纯文本语言模型用于视觉领域,使其能处理和生成任意交错的图像-文本数据。所提出方法利用了从大规模纯文本预训练中学到的语言模型的能力,如上下文学习和自由格式文本生成。保持语言模型的冻结,并对输入和输出线性层进行微调,以实现跨模态的交互。这使得该模型能处理任意交错的图像-文本输入,并生成与检索图像交错的自由格式文本。在诸如上下文图像检索和多模态对话等 grounded 任务上取得了强大的零样本性能,并展示了引人注目的交互能力。该方法适用于任意现有的语言模型,并为在视觉 grounded 设置中利用预训练的语言模型的有效、通用解决方案铺平了道路。
We propose an efficient method to ground pretrained text-only language models to the visual domain, enabling them to process and generate arbitrarily interleaved image-and-text data. Our method leverages the abilities of language models learnt from large scale text-only pretraining, such as in-context learning and free-form text generation. We keep the language model frozen, and finetune input and output linear layers to enable cross-modality interactions. This allows our model to process arbitrarily interleaved image-and-text inputs, and generate free-form text interleaved with retrieved images. We achieve strong zero-shot performance on grounded tasks such as contextual image retrieval and multimodal dialogue, and showcase compelling interactive abilities. Our approach works with any off-the-shelf language model and paves the way towards an effective, general solution for leveraging pretrained language models in visually grounded settings.
https://arxiv.org/abs/2301.13823
5、[LG] Mathematical Capabilities of ChatGPT
S Frieder, L Pinchetti, R Griffiths, T Salvatori, T Lukasiewicz, P C Petersen, A Chevalier, J Berner
[University of Oxford & University of Cambridge & University of Vienna]
ChatGPT的数学能力
要点:
-
引入一个新的数据集 GHOSTS,以涵盖研究生水平的数学,并提供语言模型数学能力的全面概括; -
将 ChatGPT 在 GHOSTS 上进行基准测试,并根据细粒度标准评估性能; -
识别ChatGPT的故障模式及其功能的局限。
一句话总结:
研究了 ChatGPT 的数学能力,引入新的数据集 GHOSTS,根据细粒度标准对 ChatGPT 进行基准测试,结论是 ChatGPT 的数学能力明显低于一般的数学研究生。
摘要:
本文通过在公开数据集和手工制作的数据集上测试 ChatGPT 的数学能力,并将其性能与在数学语料库上训练的其他模型(如Minerva)进行比较。本文还通过模拟数学家日常职业活动中出现的各种用例(回答问题、搜索定理)来测试 ChatGPT 是否能成为专业数学家的有用助手。与形式数学不同的是,形式数学有大型的形式证明数据库(如 Lean Mathematical Library),而目前用于衡量语言模型的自然语言数学数据集只包括初等数学。本文通过引入一个新的数据集 GHOSTS 来解决该问题,这是第一个由数学领域的工作研究人员制作和策划的自然语言数据集,(1) 旨在涵盖研究生水平的数学,(2) 提供语言模型数学能力的全面概括。本文在 GHOSTS 上对 ChatGPT 进行了基准测试,并根据细化的标准评估其性能。与媒体的许多正面报道相反(可能存在选择性偏差),ChatGPT 的数学能力明显低于普通数学研究生的能力。结果显示,ChatGPT 经常能理解问题,但不能提供正确的解答。
We investigate the mathematical capabilities of ChatGPT by testing it on publicly available datasets, as well as hand-crafted ones, and measuring its performance against other models trained on a mathematical corpus, such as Minerva. We also test whether ChatGPT can be a useful assistant to professional mathematicians by emulating various use cases that come up in the daily professional activities of mathematicians (question answering, theorem searching). In contrast to formal mathematics, where large databases of formal proofs are available (e.g., the Lean Mathematical Library), current datasets of natural-language mathematics, used to benchmark language models, only cover elementary mathematics. We address this issue by introducing a new dataset: GHOSTS. It is the first natural-language dataset made and curated by working researchers in mathematics that (1) aims to cover graduate-level mathematics and (2) provides a holistic overview of the mathematical capabilities of language models. We benchmark ChatGPT on GHOSTS and evaluate performance against fine-grained criteria. We make this new dataset publicly available to assist a community-driven comparison of ChatGPT with (future) large language models in terms of advanced mathematical comprehension. We conclude that contrary to many positive reports in the media (a potential case of selection bias), ChatGPT’s mathematical abilities are significantly below those of an average mathematics graduate student. Our results show that ChatGPT often understands the question but fails to provide correct solutions. Hence, if your goal is to use it to pass a university exam, you would be better off copying from your average peer!
https://arxiv.org/abs/2301.13867
另外几篇值得关注的论文:
[CL] Faithful Chain-of-Thought Reasoning
Q Lyu, S Havaldar, A Stein, L Zhang, D Rao, E Wong, M Apidianaki, C Callison-Burch
[University of Pennsylvania]
忠实思维链推理
要点:
-
提出忠实思维链(Faithful CoT)框架,将推理分解为翻译和问题解决两阶段; -
通过交织自然语言注释和可执行的符号语言程序来保证解释的忠实性; -
在10个推理数据集中的7个上取得了新的最先进的性能,显示了忠实性和准确性之间的强大协同作用。
一句话总结:
提出Faithful CoT,一种通过构建提高忠实度的推理框架,将推理任务分解为翻译(自然语言查询→符号推理链)和问题解决(推理链→答案),分别使用语言模型和确定性求解器,优于传统的 CoT 提示。
https://arxiv.org/abs/2301.13379
CL] ThoughtSource: A central hub for large language model reasoning data
S Ott, K Hebenstreit…
[Medical University of Vienna & Technical University of Denmark & Copenhagen University Hospital]
ThoughtSource: 大型语言模型推理数据集成中心
要点:
-
提出 ThoughtSource,一个元数据集和软件库,用于思维链(CoT)推理; -
ThoughtSource的目标是通过促进对CoT的定性理解,实现实证评估,并提供训练数据,来改善未来的AI系统; -
在ThoughtSource的第一个版本中,整合了6个科学/医学、3个通用领域和5个数学单词问题的回答数据集。
一句话总结:
ThoughtSource 是一个元数据集和软件库,整合了16个用于思维链(CoT)推理的数据集,以促进对 CoT 的定性理解、经验评估,并为AI系统提供训练数据。
https://arxiv.org/abs/2301.11596
[LG] Scaling laws for single-agent reinforcement learning
J Hilton, J Tang, J Schulman
[OpenAI]
单智能体强化学习的缩放律
要点:
-
在强化学习中引入了内在性能的概念,作为一个平均轮次回报的单调函数,随着模型大小和训练计算量的增加而变化; -
发现在一系列环境中的内在性能可以用模型大小和环境相互作用的幂律来衡量; -
证明了强化学习的最佳模型大小也遵循训练计算预算的幂律。
一句话总结:
提出将内在性能作为单智能体缩放率的一种方式,展示了它是如何随着模型大小和环境交互而呈幂律扩展的。
https://arxiv.org/abs/2301.13442
[CL] LongEval: Guidelines for Human Evaluation of Faithfulness in Long-form Summarization
K Krishna, E Bransom, B Kuehl, M Iyyer…
[University of Massachusetts Amherst & Allen Institute for AI]
LongEval: 长式摘要忠实度人工评估指南
要点:
-
提出LONGEVAL准则,以规范对长篇摘要的人工评价; -
对当前长篇摘要人工评价实践的162篇论文进行了调查; -
在不同领域的两个数据集上对 LONGEVAL 进行经验性验证。
一句话总结:
LONGEVAL 是一套评估长式摘要忠实度的指南,在两个数据集SQuALITY和PubMed上验证,减少了细粒度标注标注者间差异,减少了标注者工作量。
https://arxiv.org/abs/2301.13298
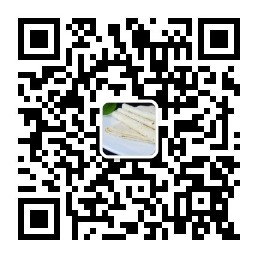